What is this specialized terminology and why should we understand it?
This term, used in specific fields, likely refers to a specialized, formally defined concept or methodology. Without further context, it's impossible to offer a definitive definition or example. However, its use in a particular domain suggests a precise meaning relevant to that discipline. For instance, it might be a technical term from engineering, a specific practice within a certain business process, or a term of art in a particular academic field.
The importance of understanding such terminology depends entirely on the context. Within a given industry or field of study, mastery of this specialized vocabulary can improve comprehension, enhance communication, and foster deeper insights. It empowers practitioners to more accurately interpret data, evaluate processes, and develop effective solutions. If this term were, for example, from a specific research area, it might be a key part of a newly defined concept or method that can be applied to future studies.
To fully understand the significance of this term, more contextual information is needed. Providing the field of application, a relevant sentence within the text it was used in, or an associated set of terms will allow for a meaningful discussion. With additional information, a more in-depth analysis and relevant explanations can be provided.
vuas
Understanding the multifaceted nature of "vuas" requires examining its core components. The following key aspects provide a framework for comprehensive analysis.
- Definition
- Application
- Methodology
- Parameters
- Metrics
- Limitations
- Validation
- Context
The term "vuas," likely an acronym or specialized terminology, demands precise definition and contextual understanding. Application in specific fields highlights its utility, while methodology underscores the approach involved. Parameters and metrics quantify its impact, and limitations acknowledge potential constraints. Validation ensures reliability and accuracy, while the broader context provides significance. Consider, for example, how a specific "vuas" application in medical imaging would differ in application and context from its use in financial modeling. Careful consideration of each aspect, individually and in combination, will yield a deeper comprehension of the terms application and significance within its field.
1. Definition
A precise definition of "vuas" is crucial for understanding its application and implications. Without a clear definition, the term becomes meaningless and its use potentially misleading. A well-defined "vuas" establishes a common understanding within a specific field, allowing for effective communication, reproducible results, and the development of consistent methodologies. A lack of clarity concerning "vuas" can lead to misinterpretations, errors in application, and ultimately, wasted resources. For example, in engineering, a poorly defined "vuas" could lead to flawed designs, whereas in financial modeling, it could result in inaccurate projections.
The importance of a rigorous definition extends to the practical application of "vuas." A standardized definition ensures consistency in measurement and analysis. This, in turn, allows for the comparison of data across different contexts and promotes a deeper understanding of the underlying phenomena being studied. If "vuas" represents a new measurement, a well-defined methodology with clearly articulated parameters is essential for replicating and validating the results. Otherwise, the reliability and validity of the "vuas" measure are compromised. For instance, in scientific research, a well-defined methodology using a clearly defined "vuas" is critical for ensuring results' reproducibility.
In summary, a definitive and well-articulated definition of "vuas" is fundamental to its proper application and interpretation. Without such clarity, the term loses meaning, and its usage in any practical context becomes problematic. This underscores the importance of precision in language and the development of standardized definitions across fields to avoid misunderstandings and ensure the reliability of any conclusions drawn from employing the term.
2. Application
The application of "vuas" depends critically on its precise definition and the specific field in which it's utilized. Effective application necessitates a clear understanding of the intended purpose, the relevant methodologies, and the potential limitations. This section explores key facets of "vuas" application, emphasizing its role and significance in various contexts.
- Data Acquisition and Processing
The application of "vuas" often involves methods for collecting and processing data. Specific instruments or procedures might be employed for data capture, transforming raw data into usable information. These procedures might include specialized sensors or algorithms tailored to specific types of data. Examples could range from sophisticated sensor networks in environmental monitoring to complex algorithms for financial data analysis. The accuracy and reliability of "vuas" heavily depend on the effectiveness and quality of the data acquisition and processing methods.
- Model Development and Validation
"Vuas" may be integrated into models designed to predict or simulate phenomena. The application of "vuas" within these models requires carefully considering how to incorporate its unique characteristics. Rigorous validation procedures are crucial to establish the model's accuracy and relevance to the intended application. Examples include simulations in engineering design, forecasting in economics, and modeling in scientific research. The effectiveness of "vuas" in these applications depends critically on the validation procedures and the model's ability to incorporate "vuas" data reliably.
- Predictive Analytics and Decision Support
In several applications, "vuas" may function as an input for predictive analytics. By using "vuas" data, insights can be generated to support decision-making processes. This can be crucial in sectors like risk management, resource allocation, or strategic planning. Examples may include using "vuas" data to predict equipment failures, assess market trends, or optimize resource allocation. The usefulness of "vuas" in this context depends on the quality of the data, the accuracy of the model, and the clarity of the decision-support framework.
- Problem Solving and Optimization
"Vuas" can play a role in problem-solving and optimization efforts. The application may focus on identifying critical factors, quantifying their impact, and exploring effective solutions. Applications might range from optimizing supply chain efficiency to streamlining manufacturing processes. Examples could be using "vuas" metrics to identify bottlenecks in operations, enabling improvements in efficiency and reducing costs. The extent to which "vuas" facilitates these endeavors depends on the problem's complexity and the available data.
These facets demonstrate the versatile application of "vuas" across diverse fields. Understanding these applications requires careful consideration of the specific context, data collection methodologies, and the integration of "vuas" into appropriate frameworks or models. The effectiveness of each application relies on the careful design and implementation of the application strategy. Ultimately, successful application of "vuas" hinges on understanding its inherent strengths and limitations within the specific context of the task or problem being addressed.
3. Methodology
The methodology employed significantly influences the validity and reliability of any analysis involving "vuas." A well-defined methodology ensures consistency, allows for reproducibility, and facilitates the comparison of results across different contexts. Crucially, the methodology dictates how "vuas" data is collected, processed, and interpreted, directly impacting the conclusions drawn. A flawed methodology can lead to spurious correlations, inaccurate interpretations, and ultimately, misleading results. For instance, in a medical study, an inconsistent methodology for collecting patient data could invalidate findings regarding "vuas" impacts on treatment outcomes.
Methodology is not merely a supplementary component but a fundamental aspect of "vuas." A robust methodology underpins the credibility and value of "vuas" application. It defines the criteria for data selection, the methods for data transformation, and the statistical approaches for analysis. The effectiveness of "vuas" in predicting future trends, optimizing processes, or identifying causal relationships hinges entirely on a sound methodological approach. In environmental science, for example, a precise methodology for monitoring pollution levels, including clear definitions of sampling procedures and data normalization processes, ensures reliable "vuas" based insights about environmental changes.
Understanding the profound connection between methodology and "vuas" is crucial. A rigorous methodology, transparently documented, enables others to critically evaluate the research process. This transparency is vital to both academic discourse and practical applications in various fields, from engineering to business analytics. Without a sound methodological framework, the meaning and impact of "vuas" remain ambiguous and unreliable. This crucial insight underscores the need for careful consideration of methodology in any endeavor leveraging "vuas" to obtain reliable results and support informed decision-making.
4. Parameters
Parameters are essential components of any analysis involving "vuas." Their precise definition and operationalization directly affect the reliability and validity of results. Appropriate parameter selection and careful consideration of their interactions are critical to drawing accurate conclusions and facilitating effective application of "vuas" in diverse contexts. Inadequate parameterization can lead to skewed interpretations, flawed predictions, and ultimately, problematic decision-making.
- Definition and Scope
Clear definitions of the parameters associated with "vuas" are fundamental. This includes explicitly stating the range of values, units of measurement, and any relevant constraints. Defining the scope of the parameters clarifies the extent of the "vuas" analysis and its applicability to different situations. For example, in a study of "vuas" effects on crop yield, parameters might include soil type, rainfall, temperature, and nutrient levels. Precisely defining these factors is critical for accurate results and meaningful interpretation.
- Measurement and Error
Reliable measurement of parameters is crucial. Errors in measurement can significantly impact the accuracy of "vuas" analysis. Sources of error, including instrument limitations, human error, and environmental factors, need careful consideration and mitigation. For instance, variations in temperature measurements during experiments could introduce errors into "vuas" results, necessitating rigorous calibration and control procedures for accurate results.
- Interactions and Dependencies
The interplay between parameters significantly influences the outcomes of "vuas" analyses. Identifying and understanding these interactions is essential for accurate predictions and effective strategies. In a financial model, the relationship between interest rates, inflation, and economic growth are critical parameters, and their interdependence must be considered. Ignoring these interactions can lead to inaccurate forecasts and suboptimal decision-making.
- Sensitivity Analysis
Sensitivity analysis evaluates the impact of changes in parameter values on the outcomes of "vuas" based models. This approach helps understand the robustness of the analysis and identify parameters that exert the most influence. For example, determining how changes in the initial conditions of a "vuas" model affect the final output provides insights into the model's stability and predictability. Sensitivity analysis illuminates the reliance of the outcome on particular parameter inputs.
In conclusion, parameters are integral to the accurate and meaningful interpretation of "vuas." Precise definitions, reliable measurements, understanding interactions, and robust sensitivity analysis are vital steps in ensuring the validity and applicability of "vuas" in various contexts. Failure to account for these aspects can undermine the credibility and reliability of any analysis involving "vuas," necessitating careful consideration of parameterization throughout the analysis process.
5. Metrics
Metrics, in the context of "vuas," are crucial for quantifying and assessing the impact, effectiveness, or outcomes associated with "vuas." A lack of appropriate metrics can obscure the true value or significance of "vuas" within a given application. Metrics serve as a critical bridge between the abstract concept of "vuas" and its tangible impact in the real world. Precisely defined metrics allow for objective evaluation and facilitate comparisons across different applications or implementations of "vuas." For instance, in a manufacturing process, "vuas" might represent a new approach to quality control. Metrics would then measure the reduction in defects, the increase in production efficiency, and the cost savings achieved.
The importance of metrics as a component of "vuas" is threefold. First, they provide a quantifiable measure of success. Without metrics, the impact of "vuas" remains anecdotal or subjective. Second, metrics allow for the tracking and monitoring of "vuas" implementation. This continuous evaluation can reveal unexpected trends or unintended consequences, enabling adjustments to the approach and optimizing effectiveness. Third, metrics enable comparisons between different implementations or variations of "vuas." This comparative analysis leads to the identification of best practices and the refinement of methodologies over time. For example, in a medical study evaluating the efficacy of a new drug using "vuas" analysis, metrics might track patient recovery rates, side effects, and overall treatment outcomes. Analysis of these metrics can help determine whether the drug warrants further clinical trials. Furthermore, metrics can be used to compare the effectiveness of the "vuas" approach against traditional methods.
In summary, metrics are indispensable for understanding and evaluating the tangible impact of "vuas." By quantifying results, facilitating continuous monitoring, and enabling comparisons, metrics transform the abstract concept of "vuas" into a concrete and measurable reality. This process not only allows for a more rigorous assessment of the value of "vuas" but also for the iterative improvement and optimization of its implementation in various domains. Carefully defined metrics are essential for justifying the adoption of "vuas" in any field and for guiding future development. The absence of appropriate metrics significantly undermines the potential of "vuas" to achieve its desired goals, and in many cases, makes meaningful conclusions virtually impossible.
6. Limitations
Any application of "vuas," like any complex methodology, encounters inherent limitations. Understanding these constraints is crucial for realistic expectations and effective implementation. Failing to acknowledge these limitations can lead to misinterpretations, inaccurate predictions, and ultimately, flawed decisions. This section explores key areas where limitations impinge upon "vuas" application and interpretation.
- Data Dependency and Quality
The accuracy and reliability of "vuas" are intrinsically linked to the quality and quantity of input data. Inadequate data, either incomplete or flawed, can lead to inaccurate conclusions or misleading predictions. This limitation requires careful data collection procedures, validation processes, and appropriate statistical techniques to address potential biases or errors. A model using "vuas" to predict market trends, for example, will be severely impacted if the underlying data contains systematic errors or is incomplete.
- Model Assumptions and Simplifications
Models incorporating "vuas" often rely on simplifying assumptions to make calculations feasible. These simplifications can introduce errors and limit the model's applicability to complex real-world scenarios. Ignoring these inherent simplifications can lead to inaccurate results, especially when dealing with highly complex systems. A model using "vuas" for environmental simulations, for example, will only be as accurate as the simplifying assumptions it employs about the system. These assumptions can limit predictive power.
- Computational Resources and Time Constraints
Complex "vuas" analyses can demand substantial computational resources and time. These resources might not always be readily available or feasible, especially when dealing with large datasets or highly sophisticated models. The limitations imposed by computational power and available time significantly restrict the scale and complexity of feasible "vuas" studies. For instance, a large-scale study employing "vuas" to analyze global climate patterns might be computationally intensive and time-consuming, impacting the speed at which results become available.
- Interpretation and Contextualization
Interpreting the outputs of "vuas" analyses requires careful consideration of the context in which the analysis is performed. Without understanding the specific limitations of the approach within the context of the application, misinterpretations or inappropriate conclusions can arise. Applying "vuas" to a diverse range of settings may reveal context-dependent limitations and necessitate adjustments in interpretation. For instance, a "vuas" model showing a predicted rise in demand for a product might be misleading if the analysis fails to consider the changing economic conditions in a specific region.
Recognizing these limitations is crucial to effectively evaluating the application and results of "vuas." A balanced assessment, acknowledging potential biases, and carefully considering the context, enables appropriate interpretation and strategic decision-making. Any application of "vuas" must acknowledge these inherent boundaries and adapt the methodology to compensate where possible, or avoid deployment where limitations prove too significant for the application's needs.
7. Validation
Validation of "vuas" methodologies is paramount. The reliability and applicability of "vuas" depend critically on rigorous validation procedures. These procedures establish the accuracy, consistency, and generalizability of "vuas" results across different contexts. Without proper validation, "vuas" outputs lack credibility and cannot support informed decisions or actions. Validation demonstrates the correspondence between the theoretical model or method ("vuas") and the real-world phenomena it seeks to represent. For example, a "vuas" model aiming to predict customer behavior must be validated using actual customer data to ensure its predictive accuracy in various market conditions.
Validation processes for "vuas" often involve multiple stages. These stages typically include defining clear criteria for success, gathering data representative of diverse contexts, implementing statistical tests to assess the model's fit and accuracy, and comparing "vuas" results with existing, well-established methods or empirical data. Moreover, validation involves assessing the model's sensitivity to different parameter inputs and identifying potential sources of error. If "vuas" is used to assess a new manufacturing process, validation would encompass trials in different production environments, with different raw materials, and under diverse operational conditions. Results are then compared with established production standards and benchmarks. Robust validation ensures the models stability and predictability across varying circumstances. Without validation, conclusions drawn from "vuas" may be unreliable, leading to incorrect interpretations and poor decision-making.
Validating "vuas" methodologies is not a one-time event but an ongoing process. New data, emerging contexts, or changes in the underlying system can necessitate reassessment and refinement of validation procedures. This iterative process enhances the reliability and adaptability of "vuas" over time. The ongoing validation ensures the continued relevance and efficacy of the model, which is particularly important in dynamic environments where conditions are ever-changing. In fields like finance or epidemiology, where contexts are highly dynamic, continuous validation becomes critical to adjusting models and ensuring their accuracy as new data emerges. Ultimately, a strong emphasis on validation contributes to building confidence in the reliability and applicability of "vuas" in diverse scenarios.
8. Context
The application and interpretation of "vuas" are deeply intertwined with context. Understanding the specific circumstances surrounding its use is crucial for accurate analysis and effective implementation. Context encompasses the environment, the goals, the available data, and the intended audience. A "vuas" model designed for a specific industry or use case may not be applicable or yield meaningful results in another. The relevance of "vuas" is contingent on understanding the particular context within which it operates.
- Industry and Domain Specificity
Different industries and domains have unique characteristics and operational contexts. A "vuas" methodology designed for financial markets might not be suitable for agricultural forecasting. The specific requirements, data availability, and regulatory framework for each field influence how "vuas" are applied and interpreted. For example, the use of "vuas" in medical diagnosis will need to adhere to strict ethical and regulatory guidelines distinct from "vuas" used in engineering design.
- Data Availability and Quality
The quality and availability of relevant data significantly influence the effectiveness and interpretation of "vuas." In contexts with limited or poor data quality, "vuas" models may produce unreliable results. The volume, type, and accessibility of data dictate the feasibility and appropriateness of applying "vuas" in a given situation. A "vuas" approach that depends on extensive historical records will face limitations if such data is unavailable or incomplete. Furthermore, the data's structure and format need to align with the model's requirements.
- Goals and Objectives
The specific objectives and goals of an analysis directly impact the choice and implementation of "vuas." Different objectives might necessitate different types of models or approaches. The desired outcome of the analysis will determine the most appropriate parameters and metrics for "vuas" assessment. Consider a business seeking to optimize supply chains using "vuas." The precise details of that goal, such as the specific cost savings target, significantly impact the "vuas" application and metrics of success.
- Regulatory and Ethical Considerations
Regulatory frameworks and ethical considerations within specific contexts influence the use of "vuas." "Vuas" applications in areas like healthcare or finance will have to adhere to strict regulations. Ethical concerns regarding data privacy, bias in models, and transparency in outputs need to be addressed. A "vuas" approach that relies on sensitive data will need to comply with data protection laws, while an approach that predicts societal impacts will need to consider potential ethical implications.
In conclusion, appreciating the multifaceted nature of context is crucial for effectively leveraging "vuas." The specific domain, data availability, defined goals, and regulatory environment must all be considered when deploying and interpreting "vuas." Ignoring contextual factors can result in misinterpretations, ineffective solutions, and potentially harmful consequences. Understanding these nuances is essential for successful and responsible application of "vuas" in various fields.
Frequently Asked Questions about "Vuas"
This section addresses common queries regarding "vuas," a specialized terminology likely used in a specific field. Precise definitions and applications vary significantly depending on the domain. These answers provide general insights, but for specific contexts, consulting relevant experts is recommended.
Question 1: What does "vuas" stand for?
The precise meaning of "vuas" is indeterminate without additional context. It likely represents a specialized acronym or technical term within a particular industry or field of study. Lacking further specification, a definitive expansion or explanation is not possible.
Question 2: What is the application of "vuas"?
The application of "vuas" depends entirely on its specific definition and the relevant field. Potential applications encompass data acquisition and processing, model development, predictive analytics, and problem-solving in various domains. More information on the field of application is essential to understand how "vuas" is employed.
Question 3: What methodologies are used with "vuas"?
The specific methodologies for working with "vuas" depend on the application and the field. These methods may involve data collection procedures, data transformation techniques, statistical analyses, and the development of models or simulations. A detailed description of the methodology is necessary to understand its application within the relevant context.
Question 4: What are the limitations of "vuas"?
Limitations of "vuas" include data dependency and quality, model assumptions, computational resources, and contextual interpretation. These limitations can impact the reliability of results. Carefully considering these factors is essential for accurate interpretation and effective implementation.
Question 5: How is "vuas" validated?
Validation procedures for "vuas" methodologies typically involve defining clear success criteria, gathering relevant data, conducting statistical analysis, and comparing results with established methods or empirical data. These procedures ensure the accuracy and consistency of "vuas" applications.
In summary, understanding "vuas" requires context-specific knowledge. Without this contextual information, answers to general queries remain incomplete. Seeking detailed information within the particular field where "vuas" is used is essential for a precise understanding.
The following section delves deeper into the practical applications and theoretical underpinnings of "vuas" methodologies in various fields.
Conclusion
The exploration of "vuas" reveals a complex and multifaceted concept, heavily dependent on the specific context of its application. Key elements, including precise definition, appropriate methodology, reliable metrics, and robust validation, are fundamental for meaningful interpretations. The limitations of "vuas," stemming from data quality, model assumptions, computational constraints, and contextual variations, must be acknowledged and addressed for responsible application. Understanding the interplay of these factors is crucial for avoiding misinterpretations and ensuring the reliability of outcomes. The success of "vuas" hinges on a thorough understanding of its operational parameters and a rigorous approach to implementation, emphasizing the importance of context-dependent strategies.
Further research into "vuas" methodologies is essential to expand the breadth of understanding and applicability. Addressing the limitations through refined models, enhanced validation techniques, and expanded data sets will strengthen the foundational framework upon which "vuas" analysis is built. The implications of "vuas" are potentially significant across diverse fields. Continued study and development are necessary to unlock the full potential and ensure responsible deployment of this specialized tool or concept, ultimately benefiting informed decision-making processes and driving advancements in areas where "vuas" is applicable.
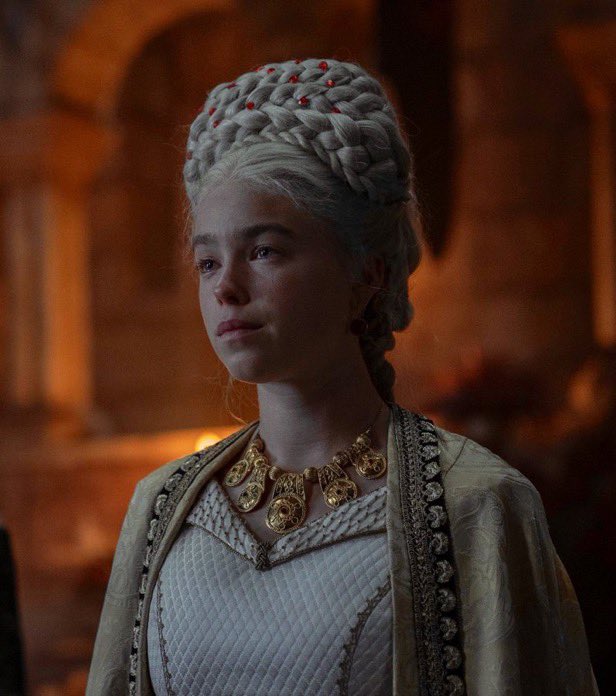

Detail Author:
- Name : Mrs. Kathryne Parisian
- Username : lauryn.hahn
- Email : davis.earlene@weber.biz
- Birthdate : 1991-09-20
- Address : 566 Era Trail New Jaidamouth, AL 23466
- Phone : 458.810.0082
- Company : Erdman, Durgan and Pollich
- Job : Legal Secretary
- Bio : Perspiciatis reiciendis dolorum natus natus sed a. Aut sapiente molestiae distinctio dolorem necessitatibus sint architecto. Recusandae neque qui unde nam ut nemo iusto.
Socials
facebook:
- url : https://facebook.com/jayne_schmidt
- username : jayne_schmidt
- bio : Animi consequatur impedit voluptatem porro.
- followers : 3839
- following : 2230
tiktok:
- url : https://tiktok.com/@jayne_schmidt
- username : jayne_schmidt
- bio : Dolorem dolores et blanditiis dignissimos qui officia magni.
- followers : 3102
- following : 1101
twitter:
- url : https://twitter.com/jayne_official
- username : jayne_official
- bio : Ea corporis vero qui earum perferendis. Qui officiis ut alias ut. Quos non maiores et. Temporibus qui libero expedita molestias praesentium est id.
- followers : 5933
- following : 2229
instagram:
- url : https://instagram.com/jayne_schmidt
- username : jayne_schmidt
- bio : Eveniet quam enim est culpa dolor. Illum qui autem pariatur unde.
- followers : 848
- following : 2159