How does real-time monitoring and analysis of transportation systems enhance efficiency and safety?
A system for tracking and analyzing the movement of vehicles and passengers in a transportation network, often utilizing sophisticated sensor technologies and data processing, provides valuable insights into traffic patterns, potential bottlenecks, and overall system performance. This information is instrumental in optimizing routes, managing capacity, and reducing delays, ultimately improving the travel experience. Examples include tracking buses, trains, or even individual cars to identify congestion, predict delays, and reroute traffic more efficiently.
Real-time monitoring and analysis in transportation systems is crucial for several reasons. It fosters a safer environment by enabling the prompt identification and resolution of potential hazards and incidents. Improved safety leads to reduced accidents and injuries. Furthermore, efficiency gains result from proactive identification of traffic flow bottlenecks, allowing for timely intervention and route adjustments. Reduced delays lead to considerable savings in travel time and fuel consumption, directly impacting both individual commuters and broader economic productivity. Historical context reveals that the evolution of this technology demonstrates a clear trend toward safer, more efficient, and more sustainable transportation systems.
Moving forward, this article will delve into the specific details of transportation management systems and highlight innovative approaches to enhance these real-time monitoring and analysis capabilities.
Real-Time Transportation Analysis
Effective transportation management hinges on comprehensive analysis of real-time data. Accurate and timely insights are critical for optimizing routes, predicting delays, and ensuring efficient operations.
- Data Collection
- Pattern Recognition
- Predictive Modeling
- Route Optimization
- Capacity Management
- Incident Response
- Performance Evaluation
- System Integration
These key aspects are interconnected. Data collection forms the foundation, enabling pattern recognition to identify trends and potential issues. Predictive models then forecast future conditions, allowing for proactive route optimization and capacity adjustments. Real-time incident response is facilitated by immediate access to data, enabling swift resolution of problems. The performance evaluation of the system is crucial for identifying areas for improvement and fine-tuning strategies. The integration of different transportation modes is essential to maximize the system's overall efficiency and effectiveness. For instance, a system tracking bus routes can integrate data from traffic sensors and even individual passenger apps, yielding comprehensive insights and ultimately improving the user experience.
1. Data Collection
Accurate and comprehensive data collection is fundamental to effective transportation management, forming the bedrock upon which real-time analyses (often referred to as 'radar mta') are built. The reliability and granularity of data directly influence the accuracy and value of subsequent analyses. Without robust data collection procedures, insights derived from transportation systems become unreliable and ultimately less effective.
Consider a city's public transportation system. Precise data on bus locations, passenger counts, and traffic conditions are essential for real-time route optimization. Delays in one area can be detected immediately, allowing for diversion of buses to less congested routes. This proactive adjustment, based on data, prevents widespread delays and enhances the overall system's efficiency. Similarly, accurate data on pedestrian traffic can inform decisions about traffic light timing, optimizing flow for both vehicles and pedestrians. Data from various sourcesGPS tracking, traffic sensors, and passenger applicationsare integrated to provide a holistic view of the system's performance. Data collection, therefore, is not merely a preliminary step, but an integral component of the 'radar mta' system, enabling real-time adjustment and informed decision-making.
The significance of data collection in transportation extends beyond immediate benefits. By understanding the patterns and trends revealed through data, cities and transportation agencies can make long-term investments in infrastructure and planning. Furthermore, collected data provides valuable benchmarks for evaluating system performance over time, allowing for continuous improvement and adaptation to changing conditions. This detailed, accurate data collection and analysis empower decision-makers with critical information for effective and responsive transportation management.
2. Pattern Recognition
Pattern recognition is a critical component of real-time transportation analysis. The ability to identify recurring patterns in traffic flow, passenger movement, and system performance is essential for proactively addressing potential issues and optimizing overall efficiency. This facet, deeply intertwined with real-time monitoring and analysis, allows for the anticipation of congestion points, the prediction of delays, and the adaptation of routes in response to emerging trends. Effective pattern recognition is the backbone of a responsive transportation management system.
- Identifying Congestion Hotspots
Analyzing historical data reveals recurring congestion points. Identifying patterns in traffic volume and speed, coupled with location data, allows for the pinpointing of specific areas prone to delays. This information is invaluable for deploying resources to address congestion proactively, potentially adjusting traffic signals, providing real-time rerouting suggestions to drivers, or implementing traffic management strategies to mitigate the impact of predictable congestion.
- Predicting Demand Fluctuations
By recognizing patterns in passenger volume across various routes and times of day, transportation systems can predict future demand. This includes recognizing seasonal patterns, special events, or even daily commute patterns. Anticipating demand allows for the dynamic allocation of resources, such as adjusting bus schedules, train frequency, or even reallocating public transit vehicles to meet anticipated needs.
- Detecting and Responding to Incidents
Identifying unusual patterns in traffic flow, such as sudden deceleration or significant deviations from typical movement, can indicate accidents or incidents. This type of pattern recognition allows for rapid response protocols to be initiated. Emergency vehicles can be routed more efficiently, alternative routes can be suggested in real-time, and system alerts can warn travelers of potential disruptions, thus minimizing the negative impact of unplanned events.
- Optimizing Resource Allocation
Identifying patterns in resource utilization such as bus occupancy rates, train passenger load, or even maintenance needs allows for proactive resource allocation. This can involve rerouting vehicles to areas of higher demand, scheduling maintenance during off-peak hours, or adjusting staffing levels in response to observed trends. Optimizing resource allocation enhances efficiency and minimizes the need for reactive measures, yielding cost savings and improved service quality.
In summary, pattern recognition is integral to "radar mta," providing the foundation for proactive management. By analyzing data, anticipating issues, and optimizing resource allocation, effective pattern recognition facilitates a more efficient, reliable, and responsive transportation system.
3. Predictive Modeling
Predictive modeling plays a pivotal role in real-time transportation analysis, functioning as a crucial component of "radar mta" systems. The application of predictive models allows for the anticipation of future conditions, thereby enabling proactive adjustments and optimized resource allocation. This anticipatory capability is essential for mitigating delays, managing congestion, and enhancing the overall efficiency of transportation networks. For instance, predictive models can forecast traffic congestion based on historical patterns, current traffic conditions, and real-time events, enabling dynamic routing adjustments for vehicles.
Real-world applications of predictive modeling within transportation networks are numerous. Consider a city's public transportation system. By analyzing historical data on ridership, weather patterns, and special events, predictive models can project potential peak demand periods. Anticipating these surges allows transportation authorities to adjust schedules, increase fleet size, or deploy additional personnel proactively, thereby minimizing delays and ensuring reliable service. Moreover, predictive models can forecast the impact of incidents, such as accidents or construction, on traffic flow. This enables transportation authorities to reroute vehicles, offer alternative transportation options, and disseminate timely alerts to commuters, preventing widespread disruption. Similarly, freight logistics operations can leverage predictive modeling to anticipate potential delays due to weather or road closures, optimizing delivery schedules and minimizing disruptions to supply chains. The accuracy and efficacy of these models are directly tied to the quality and comprehensiveness of the data utilized for training and validation, thus emphasizing the importance of consistent and reliable data collection methodologies.
In conclusion, predictive modeling is an indispensable tool for "radar mta." By enabling the anticipation of future events and the proactive adaptation of transportation systems, predictive models enhance efficiency, reliability, and responsiveness. The accurate prediction of congestion, the anticipation of demand surges, and the prompt response to incidents contribute to a more efficient and user-friendly transportation experience. However, the successful implementation of predictive modeling necessitates robust data infrastructure and ongoing refinement of models based on evolving patterns, effectively ensuring continued adaptation to changing conditions.
4. Route Optimization
Route optimization, a key element in effective transportation management, is intrinsically linked to radar mta systems. Real-time data analysis, a defining characteristic of radar mta, provides the foundation for dynamic route adjustments. Leveraging this data enables systems to adapt to changing conditions, minimizing delays and maximizing efficiency in the movement of people and goods.
- Dynamic Rerouting Strategies
Route optimization, empowered by real-time data, allows for dynamic rerouting. Sensors and GPS tracking data provide instantaneous updates on traffic conditions, enabling the system to identify and react to congestion points. This means adjusting routes in real-time to circumvent delays, optimizing the journey for all users. For example, during an unexpected road closure, the system could automatically reroute vehicles to alternative routes, minimizing travel time and avoiding delays. This dynamic capability is a significant advantage over static route planning, offering enhanced responsiveness and flexibility.
- Real-Time Traffic Flow Adjustments
Systems using radar mta can analyze real-time traffic flow data. By monitoring traffic density, speed, and patterns, the system can predict and react to congestion, optimizing routes in real-time. The system might adjust traffic signal timing, suggest alternative routes to drivers, or even optimize the scheduling of public transportation, dynamically responding to changing traffic patterns. Such adjustments contribute to a more fluid and efficient traffic flow.
- Predictive Route Optimization
By incorporating predictive modeling into radar mta systems, route optimization becomes proactive rather than reactive. The system anticipates future traffic conditions based on historical data, real-time information, and external factors such as weather events. For instance, anticipating a rush hour bottleneck allows for preemptive adjustments in routes and schedules, mitigating delays before they occur. This anticipatory approach results in optimized travel times and improved service reliability for users.
- Integration with Transportation Modes
Optimizing routes is significantly enhanced when considering diverse transportation modes. Radar mta systems can integrate data from various sources, including public transit, ride-sharing platforms, and individual vehicle tracking. This integrated approach enables a comprehensive view of the entire transportation network, allowing for optimized intermodal routes and seamless transitions between different modes of transport. For example, rerouting a bus due to a road closure might be coupled with suggestions for alternative ride-sharing options or public transportation connections, offering users multiple optimized alternatives.
In essence, route optimization, when powered by real-time data analysis, becomes a dynamic and responsive process. This capability, deeply rooted in radar mta, results in reduced delays, improved traffic flow, optimized resource utilization, and a superior overall transportation experience for all users. The optimization isn't simply about adjusting current routes; it's about intelligently and proactively managing the entire transportation network to provide the most efficient and effective options for all travelers.
5. Capacity Management
Effective capacity management is inextricably linked to radar mta systems. Radar mta systems provide the real-time data necessary for accurate capacity assessments, enabling proactive adjustments to optimize resource allocation and prevent bottlenecks. This dynamic adaptation is crucial for maintaining efficient and reliable transportation operations. Without the real-time insights afforded by radar mta, capacity management becomes significantly more reactive and susceptible to breakdowns, especially during periods of peak demand or unforeseen incidents. For instance, a transit agency monitoring bus routes in real time can adjust schedules based on current passenger loads and traffic conditions, preventing overcrowding on certain lines and ensuring a smooth flow of passengers.
The practical significance of this connection is evident in numerous scenarios. During rush hour, a radar mta system can track passenger loads on different bus routes, allowing the system to reroute buses to less congested areas or adjust schedules to meet demand more effectively. In freight logistics, radar mta allows monitoring of transport vehicles and cargo, ensuring efficient utilization of available capacity. This prevents delays caused by unexpected congestion or overload. Further, it facilitates the allocation of resources such as additional personnel or vehicles in areas experiencing high demand. Similarly, air traffic control systems utilize similar data to manage air traffic density and prevent mid-air collisions, optimizing air space utilization. These examples highlight how radar mta enhances capacity management, providing actionable intelligence for optimizing resource deployment and preventing disruptions.
In conclusion, capacity management critically benefits from the real-time data and analysis provided by radar mta systems. The integration of real-time information allows for dynamic adjustments in resource allocation, leading to increased efficiency, improved reliability, and a smoother overall experience for users. Accurate capacity assessment, enabled by radar mta, prevents bottlenecks and ensures systems can handle peak demand or unforeseen events. By understanding the interconnection between capacity management and radar mta, transportation authorities and managers can make informed decisions, maximizing the value of their systems and improving the experience of users across various transport sectors.
6. Incident Response
Effective incident response is a critical component of any robust radar mta system. The real-time data analysis capabilities of radar mta systems are instrumental in enabling swift and informed responses to disruptions, accidents, or other unexpected events. The connection lies in the ability of radar mta to identify anomalies in real-time, enabling the rapid dissemination of information to relevant stakeholders and triggering pre-defined response protocols. This timely intervention can significantly mitigate the negative impacts of incidents, minimizing delays, disruptions, and potential hazards.
Consider a major traffic accident on a highway. Real-time data from sensors and vehicle tracking systems, components of a radar mta system, can immediately identify a significant decrease in traffic flow at a specific location. This information, disseminated quickly, allows authorities to initiate rerouting strategies, deploy emergency personnel, and disseminate alerts to motorists. Without the real-time insights provided by a radar mta system, response time would likely be significantly delayed, exacerbating the consequences of the incident. Similar principles apply to public transit systems, where real-time tracking of buses and trains can quickly identify breakdowns, allowing for proactive rerouting and minimizing delays for passengers. In a rail network, real-time monitoring can pinpoint signal failures, facilitating a swift response and preventing potential collisions. The prompt and targeted interventions, facilitated by radar mta, directly translate to reduced disruptions and improved safety.
The significance of incident response within radar mta systems lies in its ability to enhance safety and efficiency. By providing a rapid and intelligent response to incidents, these systems safeguard critical infrastructure, reduce delays, and prevent escalation of issues. Prompt and accurate information dissemination, enabled by real-time data analysis, is crucial in minimizing the impact of incidents and ensuring the continuity of transportation services. While challenges remain, such as ensuring data reliability and developing adaptable response protocols, the understanding of the crucial role incident response plays within radar mta systems remains indispensable for creating safer, more efficient transportation networks.
7. Performance Evaluation
Performance evaluation, a crucial aspect of any transportation system, is intricately linked with radar mta. The real-time data and analysis capabilities of radar mta systems provide the essential foundation for assessing and improving system performance. Thorough evaluation, informed by this data, allows for the identification of areas needing improvement, the optimization of existing strategies, and the development of future enhancements within the broader transportation network.
- Identifying Bottlenecks and Congestion Points
Analysis of real-time traffic data allows for the precise identification of congestion hotspots. By observing patterns and fluctuations in traffic flow, radar mta systems can pinpoint areas where delays are consistently occurring. This granular insight enables targeted interventions, such as adjusting traffic signal timing or implementing dynamic routing strategies, leading to improved efficiency and reduced congestion.
- Assessing System Reliability and Response Time
Radar mta's constant monitoring of system performance metrics, such as travel times and response times to incidents, offers a clear picture of reliability. Evaluation of this data reveals potential vulnerabilities and areas requiring enhancement. For example, if average travel times consistently exceed projected values during peak hours, radar mta data can pinpoint the root cause, guiding adjustments to optimize the system's performance during high-demand periods.
- Evaluating Resource Utilization and Efficiency
Radar mta systems can track and measure resource utilization, such as vehicle occupancy rates, fuel consumption, and personnel deployment. By evaluating these parameters, the efficiency of resource allocation can be ascertained. Discrepancies between projected and actual resource consumption reveal areas needing optimization. For example, if bus routes consistently exhibit underutilized capacity, adjustments to scheduling or route optimization can improve efficiency and resource management.
- Measuring User Satisfaction and Experience
Data captured through passenger feedback systems, integrated with radar mta data, allows for the assessment of user satisfaction. Monitoring key metrics such as travel time, delays, and perceived wait times helps identify areas where the user experience could be improved. Analyzing these data points allows for adjustments to service offerings, schedules, or infrastructure improvements to enhance the overall user experience.
In essence, performance evaluation, informed by the real-time data and analysis of radar mta systems, fosters a proactive and data-driven approach to transportation management. The insights gleaned from these systems support the continuous refinement and improvement of transportation networks, ultimately optimizing efficiency, safety, and the overall user experience. This cycle of evaluation and adjustment, supported by the continuous flow of information from radar mta, is crucial for the long-term sustainability and effectiveness of any modern transportation infrastructure.
8. System Integration
System integration is fundamental to the effectiveness of radar mta systems. A fully integrated system, encompassing diverse data sources and transportation modes, is crucial for a comprehensive and actionable understanding of the entire network. A disparate collection of isolated systems, each providing partial insights, lacks the holistic perspective necessary for effective management and optimization. Successfully integrated systems allow for seamless data exchange, facilitating a real-time, comprehensive view of traffic patterns, passenger flows, and potential bottlenecks across the entire network, from individual vehicles to public transit.
The significance of integration is exemplified in various applications. A system tracking public buses must integrate with traffic data from sensors, GPS data from individual vehicles, and potentially even real-time passenger information from mobile applications. This interconnectedness allows for dynamic route adjustments based on real-time traffic conditions, potentially rerouting buses to avoid congested areas. Similarly, in freight logistics, integrating data from various delivery vehicles, warehouses, and shipping ports allows for real-time tracking of cargo, enabling proactive management of potential delays and optimizing delivery schedules. Such integrations enable a holistic view of the entire transportation chain, from origin to destination, enhancing efficiency and minimizing disruptions. Without such integration, the insights from radar mta systems become fragmented and less impactful, ultimately diminishing their value in optimizing the overall transportation system.
In conclusion, system integration is not merely a component of radar mta; it is a prerequisite for its effectiveness. The ability to consolidate data from diverse sources into a unified platform enhances the comprehensiveness and accuracy of analyses. This holistic view is essential for developing proactive solutions and managing complex transportation systems. However, achieving seamless integration faces challenges, such as compatibility issues between various data formats and systems, as well as the need for robust security protocols to safeguard sensitive data. Overcoming these challenges ensures the full realization of the potential of radar mta systems for efficient and reliable transportation networks.
Frequently Asked Questions
This section addresses common inquiries about radar MTA, a system for real-time monitoring and analysis of transportation systems. Clarity and accurate information are paramount in understanding the intricacies of this technology.
Question 1: What is radar MTA, and how does it differ from traditional methods of transportation management?
Radar MTA signifies real-time monitoring and analysis for transportation systems. Unlike traditional methods, which often rely on static data or periodic updates, radar MTA leverages constant data streams from various sourcessensors, GPS, and passenger appsto provide a dynamic, ever-evolving picture of the transportation network. This allows for more immediate identification of issues and proactive adjustments, leading to greater efficiency and reduced delays.
Question 2: What are the key benefits of implementing a radar MTA system?
Implementation of radar MTA systems yields enhanced safety by enabling prompt identification and resolution of potential hazards. Efficiency gains result from proactive identification of bottlenecks, facilitating timely interventions and route adjustments. Furthermore, it leads to cost savings via reduced delays and optimized fuel consumption.
Question 3: What types of data does a radar MTA system collect?
Radar MTA systems gather diverse data, encompassing vehicle locations, passenger loads, traffic conditions, and weather patterns. This data is often drawn from a variety of sources, including traffic sensors, GPS units, and passenger apps. The specific data collected varies depending on the system's design and the goals of the transportation organization.
Question 4: How does radar MTA improve the user experience for commuters?
Radar MTA enhances the commuter experience by offering real-time information about transportation options. It facilitates informed decisions on route selection and provides up-to-date information about potential delays, thus minimizing disruptions to the journey. This accessibility and proactiveness ultimately improve satisfaction and trust in the system.
Question 5: What are the potential challenges associated with implementing a radar MTA system?
Implementing radar MTA systems presents challenges, including system integration across various platforms, ensuring data accuracy and reliability, and addressing potential privacy concerns. The significant investment in technology and training personnel are also crucial aspects of a successful implementation.
Understanding these FAQs provides a clearer comprehension of the scope and implications of radar MTA. Continued research and development are essential to overcome challenges and unlock the full potential of radar MTA systems for optimized transportation management.
The subsequent section will delve into specific case studies and technological advancements in radar MTA systems.
Conclusion
This article has explored the multifaceted nature of radar MTA systems, highlighting their crucial role in modern transportation management. Key aspects examined include data collection and analysis methodologies, the development of predictive models, route optimization strategies, capacity management techniques, and effective incident response mechanisms. The integration of diverse data sources, including real-time traffic, passenger information, and weather patterns, has been shown to significantly enhance the responsiveness and efficiency of transportation systems. The transformative potential of radar MTA has been emphasized, demonstrating its ability to streamline operations, mitigate disruptions, and enhance the overall user experience. Radar MTA systems facilitate a more adaptable and dynamic approach to managing transportation networks, allowing for proactive adjustments to evolving conditions.
Moving forward, the continued development and application of radar MTA technologies are crucial for addressing the complex challenges of modern transportation. Further research and investment in data infrastructure and analytical tools are essential to optimize performance, enhance safety, and improve the overall efficiency of transportation systems. The integration of innovative technologies, such as artificial intelligence and machine learning, holds further potential to enhance the predictive capabilities of radar MTA systems, facilitating even more sophisticated and responsive transportation management. Ultimately, the success of future transportation systems hinges on the continued advancement and responsible application of radar MTA technologies.
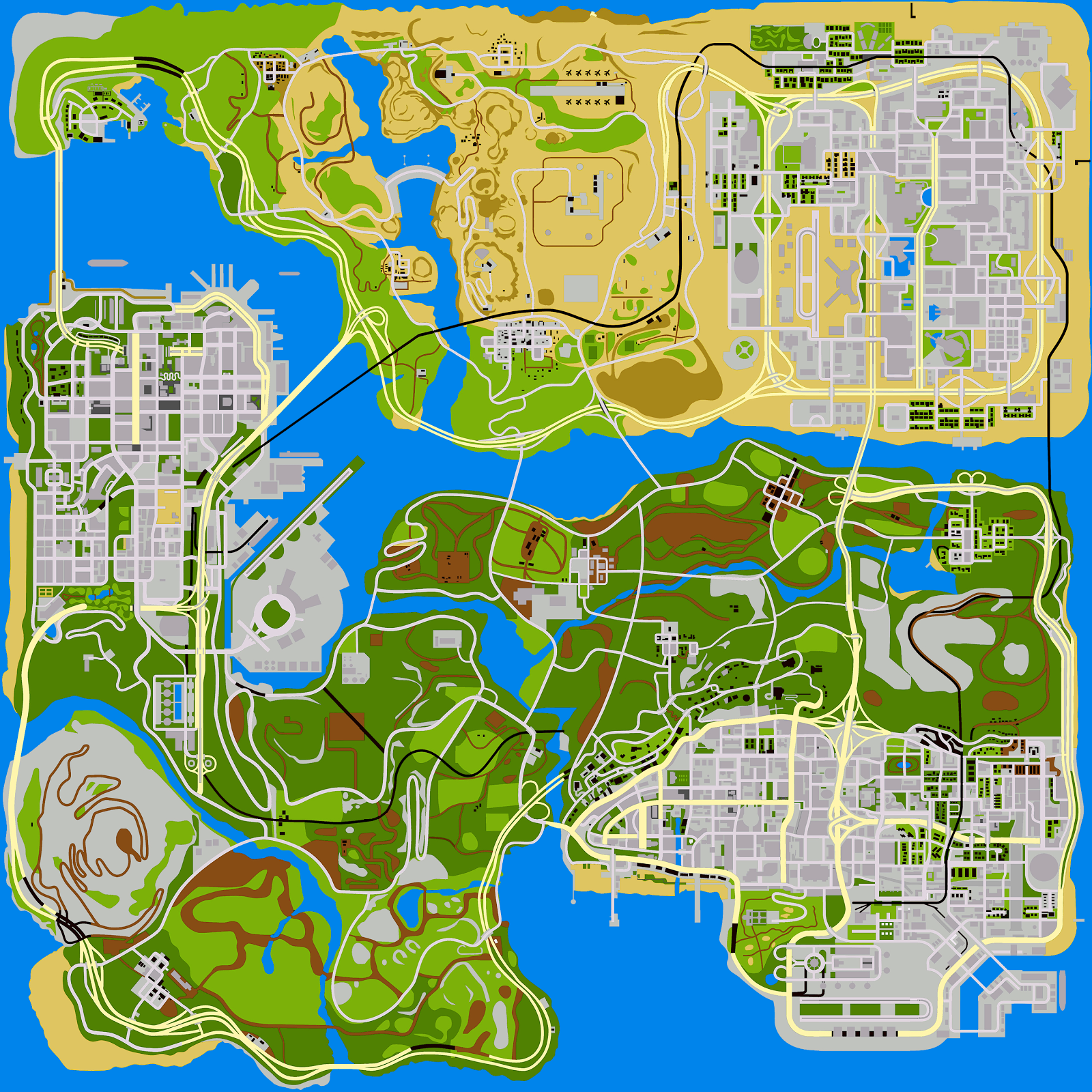
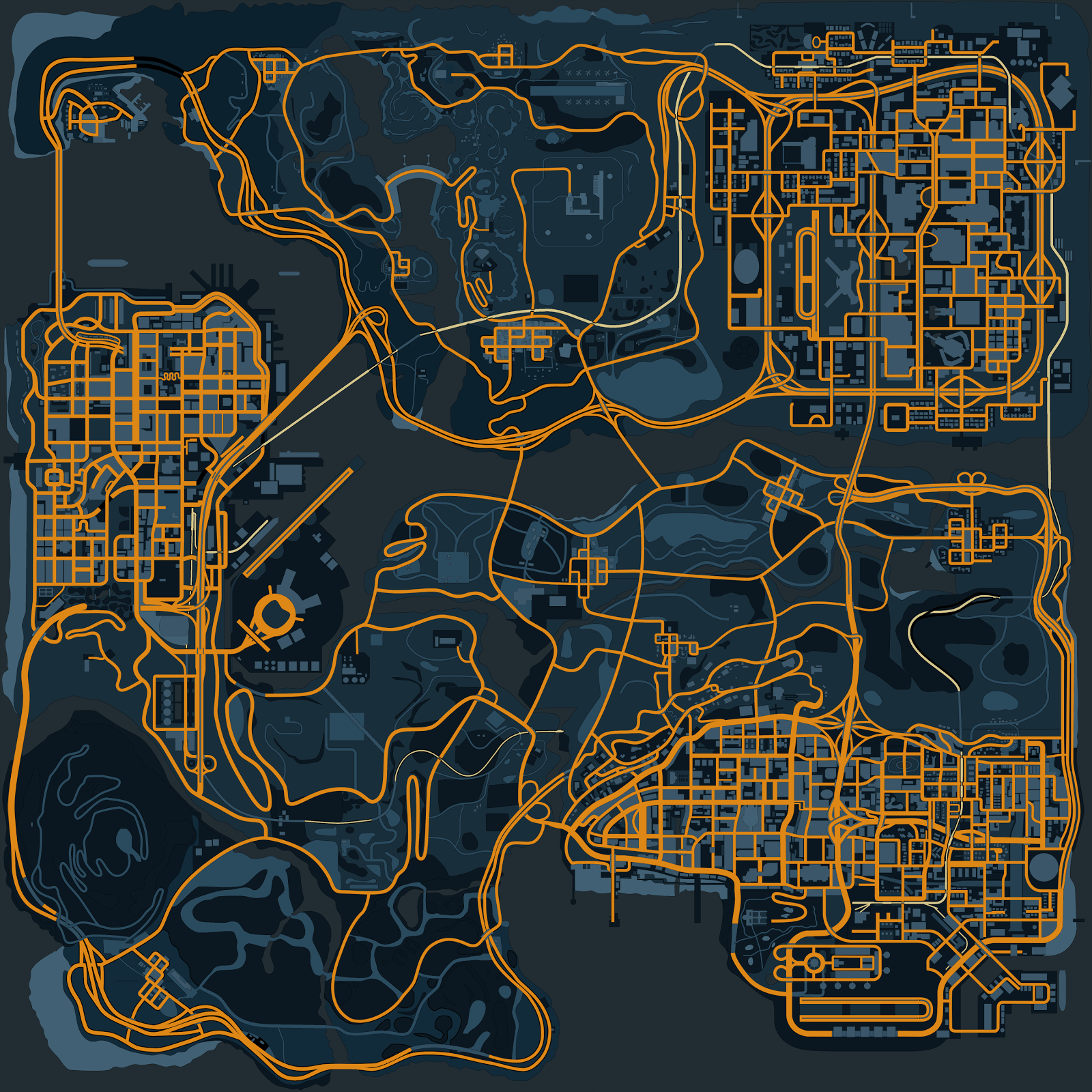
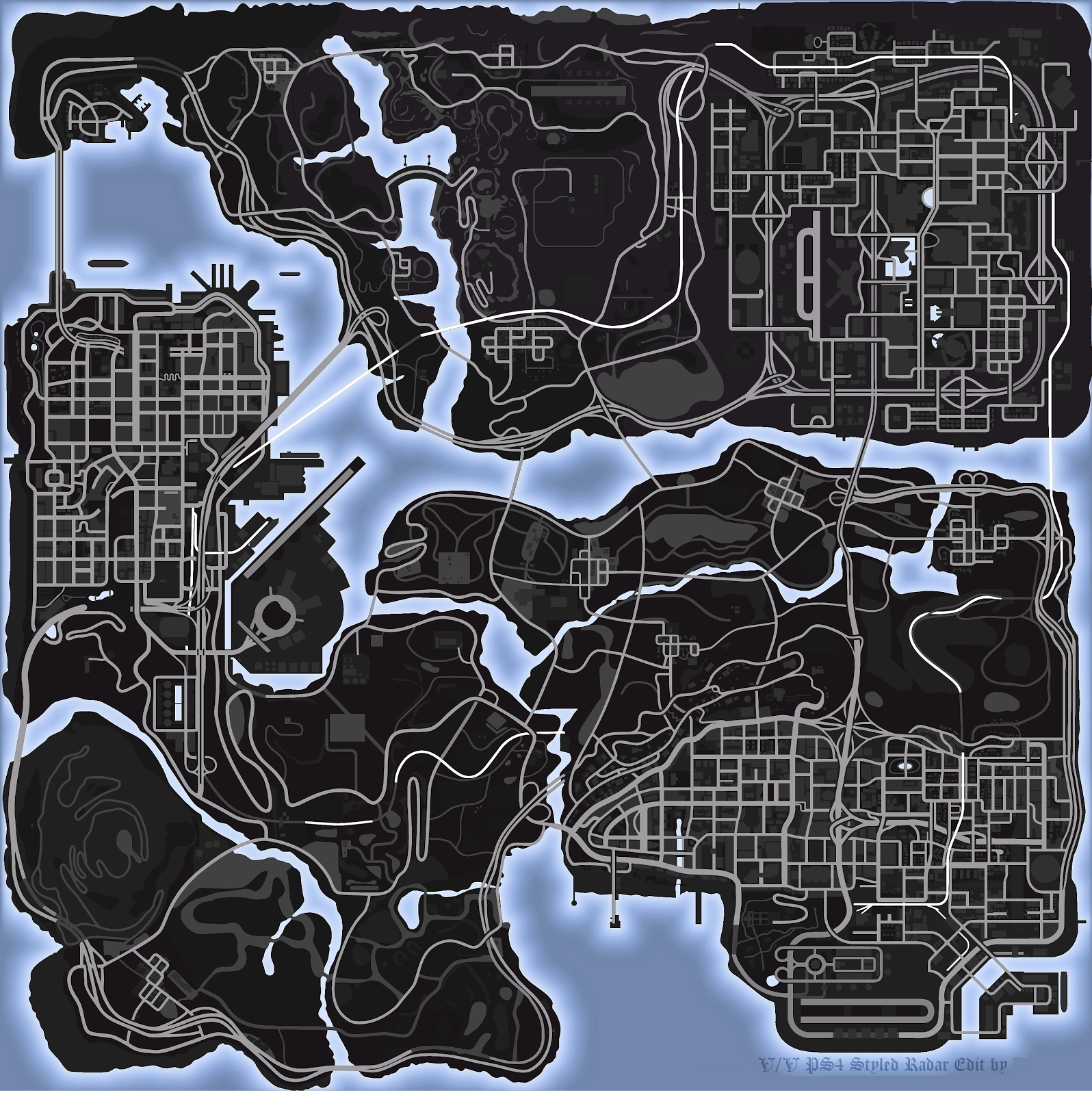
Detail Author:
- Name : Verla Lubowitz
- Username : acummings
- Email : bethany.goodwin@mosciski.biz
- Birthdate : 1975-06-05
- Address : 22644 Sylvan Forest Suite 297 New Coltonburgh, AK 01289-0039
- Phone : 786-475-1155
- Company : Satterfield-Schmitt
- Job : Instructional Coordinator
- Bio : Qui perferendis voluptatem quos corrupti. Eos ratione et nemo possimus. Eum quidem et natus tempora consequatur modi quia.
Socials
instagram:
- url : https://instagram.com/warren_predovic
- username : warren_predovic
- bio : Libero eligendi consectetur nostrum. In quam corporis laborum non dolor ex veritatis.
- followers : 6275
- following : 1415
facebook:
- url : https://facebook.com/warren1522
- username : warren1522
- bio : Labore iste officiis at ut quisquam corporis.
- followers : 3144
- following : 322
tiktok:
- url : https://tiktok.com/@warren1999
- username : warren1999
- bio : Cupiditate aliquid eum laborum laboriosam.
- followers : 1805
- following : 2306
linkedin:
- url : https://linkedin.com/in/wpredovic
- username : wpredovic
- bio : Sint quaerat reprehenderit sequi distinctio nisi.
- followers : 3029
- following : 1771
twitter:
- url : https://twitter.com/warren1931
- username : warren1931
- bio : Ipsum doloremque suscipit possimus quas sit modi. Dignissimos sunt iste ut est porro. Voluptatem odio eius dolores atque qui.
- followers : 6621
- following : 762