What is this complex system, and how does it contribute to modern advancements? A high-dimensional model, meticulously crafted, underpins many innovations.
A high-dimensional model (HDMM) encompasses a mathematical framework for representing and analyzing complex systems characterized by numerous variables. These systems, which can range from climate patterns to financial markets, are often too intricate to comprehend through traditional methods. This model simplifies the complexity by considering a multitude of interacting factors, enabling researchers to discover underlying patterns and relationships that may otherwise remain hidden. For example, a model might analyze consumer purchasing patterns across various demographics, products, and time periods, identifying key factors driving trends. This level of detail is often vital for developing informed strategies and predictions.
The importance of a high-dimensional model lies in its capacity to extract meaningful insights from a sea of data. This allows for better understanding and, consequently, more effective predictions in various fields, from scientific research to business strategy. HDMMs have revolutionized areas like data mining, machine learning, and artificial intelligence, fostering new levels of precision in modeling and analysis. The efficiency of these models is essential given the explosive growth of data available in the digital age.
This exploration of HDMM lays the groundwork for understanding the broader applications of advanced mathematical modeling. We will delve into particular implementations of these techniques across diverse domains, highlighting practical examples and their implications.
hdmm
High-dimensional models (HDMMs) are crucial for analyzing complex systems with numerous variables. Understanding their key aspects is essential for effective application and interpretation.
- Complexity
- Data analysis
- Pattern recognition
- Predictive capability
- Model refinement
- Interdisciplinary application
These aspects collectively define the strength of HDMMs. Complexity arises from the many variables within the system, driving the need for sophisticated analysis. Data analysis forms the foundation, extracting patterns for recognition and predictive modeling. Refining models is vital, as is the adaptability of these models to interdisciplinary fields, such as climate science or finance. Predictive capability, achieved through accurate pattern recognition, is essential for informed decision-making. For instance, a high-dimensional model predicting consumer behavior can assist in optimizing marketing strategies, showcasing the real-world applications of HDMMs.
1. Complexity
Complexity is intrinsic to many real-world phenomena, demanding specialized analytical tools. High-dimensional models (HDMMs) directly address this challenge. The inherent complexity of systems, characterized by numerous interacting variables, necessitates the use of these models. This complexity arises from interconnected relationships within systems, ranging from biological processes to economic fluctuations. For instance, the human brain, with its billions of neurons and trillions of synaptic connections, exemplifies a system characterized by substantial complexity. Analyzing such intricacy requires computational models capable of handling many variables simultaneously. HDMMs provide this capability.
The significance of complexity as a component of HDMMs stems from its direct impact on model accuracy and predictive power. Accurate representation of a system's intricate relationships is critical for realistic simulations and reliable forecasts. Consider climate modeling; the interactions among atmospheric conditions, ocean currents, and landmasses create a highly complex system. HDMMs, designed to handle this complexity, are indispensable for creating reliable climate projections. Furthermore, in finance, the complexity of market interactions and the interplay of countless variables demand HDMMs for comprehensive modeling and risk assessment. Without acknowledging and accounting for this complexity, models would struggle to capture the nuances of these systems. This in turn leads to inaccurate estimations and flawed predictions, highlighting the profound influence complexity exerts on HDMM application.
In summary, complexity is not merely a challenge for HDMMs but a defining factor. A crucial element for constructing reliable HDMMs is the precise representation of the system's complexity. Addressing this intricacy is paramount for accurate predictions and informed decision-making. Successfully navigating the complexities of systems through HDMMs unlocks the potential for advancements across diverse fields, from scientific discovery to practical applications in business and policy.
2. Data Analysis
Data analysis is fundamental to high-dimensional model (HDMM) construction and application. The volume, variety, and velocity of modern data necessitate sophisticated analytical techniques capable of handling intricate relationships. HDMMs rely on comprehensive data analysis to extract relevant patterns, identify key variables, and establish the necessary connections for accurate predictions and robust model development. Effective data analysis is not merely a precursor; it is an integral component of the HDMM framework, without which the models lose their predictive power and utility.
Real-world examples demonstrate the crucial role of data analysis within HDMMs. In financial modeling, vast datasets encompassing market trends, economic indicators, and investor behavior are crucial inputs for creating accurate predictive models. Comprehensive analysis of these datasets is essential for detecting market fluctuations, identifying risk factors, and informing investment strategies. Similarly, in climate science, analyzing historical weather patterns, satellite imagery, and atmospheric data is indispensable for constructing high-dimensional models. These models enable more precise predictions of future climate conditions, essential for adaptation strategies and mitigation efforts. The effectiveness of both financial and climate models hinges on the quality and comprehensiveness of the data analysis performed. Poor analysis leads to inaccurate predictions and potentially harmful outcomes.
Recognizing the interconnectedness between data analysis and HDMMs highlights the need for robust analytical methodologies. This necessitates techniques to effectively handle high-dimensional data, manage large datasets, and uncover complex relationships. Challenges include dealing with missing data, noisy observations, and the potential for overfitting models to training data. Consequently, developing data analysis strategies that address these limitations is critical for ensuring accurate model representations of the underlying phenomena. This underscores the importance of employing sophisticated data preprocessing methods, feature selection techniques, and model validation procedures. An understanding of this intricate relationship lays the foundation for advancing the development and application of HDMMs across a wide range of disciplines.
3. Pattern Recognition
Pattern recognition is integral to high-dimensional models (HDMMs). HDMMs aim to discern underlying structures and relationships within complex datasets. Pattern recognition forms the core of this process, enabling the identification of recurring patterns and trends within the data. These patterns, once identified, can be used to predict future behaviors and trends. Effective pattern recognition in HDMMs is crucial for building predictive models capable of handling the vast and intricate datasets typical of modern applications.
The importance of pattern recognition in HDMMs is demonstrated in various fields. Consider medical diagnosis. Analyzing patient datamedical history, symptoms, test resultsvia HDMMs with pattern recognition algorithms can identify patterns indicative of specific diseases. These patterns, gleaned from massive datasets, can assist in earlier and more accurate diagnoses, leading to improved patient outcomes. Similarly, in financial modeling, HDMMs with pattern recognition capabilities can analyze market trends, identifying patterns in price fluctuations and investor behavior. These insights facilitate informed investment decisions and risk assessment, potentially mitigating financial losses. In climate science, pattern recognition in HDMMs is used to identify recurring weather patterns, contributing to more accurate climate predictions and facilitating the development of effective strategies for climate change adaptation. These diverse examples illustrate the practical significance of this approach.
In essence, pattern recognition within HDMMs is critical for extracting meaningful insights from complex data. By identifying underlying patterns, HDMMs enable more accurate predictions and informed decision-making across numerous disciplines. The sophistication and efficiency of pattern recognition algorithms directly influence the reliability and usefulness of HDMMs. Challenges in pattern recognition include the potential for false positives, the need for large datasets, and the difficulty in distinguishing between random noise and meaningful patterns. Addressing these challenges is crucial for developing robust and reliable high-dimensional models applicable in various real-world situations.
4. Predictive capability
Predictive capability is a defining characteristic of high-dimensional models (HDMMs). The core function of an HDMM lies in its ability to forecast future outcomes based on observed data and established relationships within a complex system. This predictive power is crucial, enabling informed decision-making across various domains. The accuracy of predictions generated by HDMMs hinges on the model's capacity to capture the intricate interplay of numerous variables and historical patterns. Accurate predictions are essential for adapting to and managing complex systems effectively.
Real-world applications underscore the importance of predictive capability in HDMMs. In financial modeling, accurate predictions of market trends and potential risks are vital for investment strategies. Precise forecasting can help mitigate financial losses and optimize returns. Similarly, in healthcare, HDMMs with strong predictive capabilities can assist in identifying individuals at high risk for specific diseases, allowing for proactive interventions and preventative measures. In climate science, HDMMs enable the prediction of future climate scenarios, providing critical information for environmental policies and disaster preparedness. These applications demonstrate how predictive power, as an inherent component of HDMMs, leads to tangible benefits in various sectors.
The reliability of predictions from HDMMs hinges on several factors. The quality of input data is paramount; inaccuracies or biases in data can lead to unreliable forecasts. The complexity of the system being modeled is another factor. Highly intricate systems with numerous interdependencies might be difficult to capture effectively by the model, potentially impacting the accuracy of predictions. Furthermore, the model's assumptions and limitations need careful consideration. By understanding the inherent limitations and acknowledging potential biases, users can make more informed judgments based on HDMM predictions. Robust validation and verification procedures are vital for ensuring the reliability of predictions, fostering confidence in the outcomes generated by HDMMs and supporting sound decision-making.
5. Model Refinement
Model refinement is a crucial iterative process in the development and application of high-dimensional models (HDMMs). It involves systematically improving the accuracy and reliability of these models through adjustments, modifications, and enhancements. This ongoing process ensures models effectively represent the complexities of real-world systems, leading to more accurate predictions and informed decision-making.
- Data Integration and Enhancement
Refinement often necessitates incorporating new data, adjusting existing datasets, or addressing data inconsistencies. Modern HDMMs are often trained on large datasets; however, inaccurate or incomplete data can lead to inaccurate or biased model outputs. Improving the quality and comprehensiveness of the data used in model training is a key step in model refinement, potentially improving prediction accuracy. For instance, in financial models, incorporating updated economic indicators and market data strengthens predictive capabilities. Similarly, in climate models, integrating satellite observations and ground measurements refines the representation of atmospheric and oceanic processes.
- Algorithm Optimization
Model refinement can involve optimizing the algorithms used in HDMMs. Different algorithms exhibit varying performance characteristics when analyzing high-dimensional data. Choosing and fine-tuning algorithms can enhance efficiency and improve model performance. This involves selecting algorithms adept at handling large datasets or complex relationships within the system being modeled. For example, transitioning from linear regression to more sophisticated machine learning techniques such as neural networks can improve the accuracy and complexity of forecasts in certain applications.
- Parameter Adjustment and Validation
Refining HDMMs often involves adjusting model parameters to optimize performance and reduce errors. These parameters might encompass thresholds, weights, or other crucial settings influencing the model's behavior. Furthermore, validation processes provide critical feedback on the model's efficacy and predictive capabilities. These validations involve comparing the model's predictions against actual outcomes, allowing for adjustments to parameters and algorithms to enhance predictive power. A crucial aspect of model refinement is ongoing validation, allowing the model to be adaptable to evolving conditions.
- Model Simplification and Generalization
While HDMMs can capture intricate relationships, over-complexity can reduce interpretability and introduce computational challenges. Model refinement can involve simplifying the model by discarding less influential variables or optimizing the model's architecture, thereby enhancing its clarity and generalizability to new datasets or conditions. This involves careful evaluation of which components of the model contribute most to the output and streamlining the model to exclude redundant aspects, optimizing efficiency and comprehensibility. For instance, a refined model might better capture the essential interactions without overly complex mechanisms.
In essence, model refinement in HDMMs is a continuous process that iteratively improves the model's capability to represent the complex real-world systems it is designed to analyze. By incorporating new data, optimizing algorithms, adjusting parameters, and potentially simplifying the model, continuous refinement enhances both accuracy and practical utility, ultimately bolstering the efficacy and impact of high-dimensional models across various scientific and industrial domains.
6. Interdisciplinary application
High-dimensional models (HDMMs) derive their strength from their applicability across diverse disciplines. The ability of HDMMs to effectively analyze complex systems, characterized by numerous interacting variables, transcends disciplinary boundaries. This interdisciplinary application arises from the fundamental nature of HDMMs as analytical tools. The core principles of these models, such as data analysis, pattern recognition, and predictive modeling, are applicable to a wide spectrum of scientific, engineering, and social phenomena. Consequently, HDMMs find practical application in fields as varied as climate science, finance, and healthcare. The interconnectedness of these fields is a significant driver for HDMMs' success in real-world applications.
Real-world examples illustrate the interdisciplinary nature of HDMMs. Climate modeling, for example, necessitates understanding interactions between atmospheric, oceanic, and terrestrial systems. HDMMs can analyze vast datasets from various sources to create comprehensive models capable of predicting future climate scenarios. Similarly, financial modeling often integrates economic indicators, market data, and investor behavior. HDMMs can analyze the intricate relationships between these elements to forecast market trends and assess risks. Furthermore, healthcare applications, such as disease prediction and treatment optimization, leverage HDMMs to analyze patient data, medical history, and genetic information to predict disease progression and personalize treatments. These disparate examples demonstrate HDMMs' ability to bridge disciplinary divides, providing common analytical frameworks and promoting collaboration.
Interdisciplinary application, as a component of HDMMs, fosters innovation and knowledge synthesis. By enabling researchers and practitioners from different fields to apply similar analytical frameworks, HDMMs promote cross-disciplinary collaboration. Such collaboration accelerates advancements in knowledge and solutions. Understanding this interdisciplinary capability is crucial for realizing the full potential of HDMMs. Despite the benefits, challenges remain, particularly in ensuring data compatibility and consistency across disciplines and in developing models that accurately represent complex phenomena with significant interactions. Addressing these challenges demands interdisciplinary collaboration and dedicated efforts to develop standardized approaches. Ultimately, the success of HDMMs hinges on their broad applicability, reflecting their significance as powerful tools capable of addressing intricate, complex problems across diverse domains.
Frequently Asked Questions about High-Dimensional Models (HDMMs)
This section addresses common inquiries regarding high-dimensional models (HDMMs), offering concise and informative responses to clarify key concepts and applications.
Question 1: What are high-dimensional models (HDMMs), and why are they necessary?
High-dimensional models are mathematical frameworks designed to analyze complex systems with numerous variables. These systems, encompassing diverse fields like climate science and finance, often involve intricate interactions among numerous factors. Traditional methods may struggle to effectively model such complexities, highlighting the necessity of HDMMs to capture the nuanced relationships and interactions within these intricate systems. This enhanced capability enables more accurate predictions and informed decision-making.
Question 2: What are the key challenges associated with developing and implementing HDMMs?
Developing and implementing HDMMs presents several significant challenges. Data volume and complexity often pose substantial hurdles. Managing and processing large datasets is crucial for model accuracy, but considerable computational resources are frequently required. Addressing potential biases and inaccuracies in the data used for model training is equally important for producing robust and trustworthy models. Furthermore, understanding and interpreting the output of complex models requires specialized expertise.
Question 3: What are the common applications of HDMMs?
HDMMs find widespread application across disciplines. In finance, they can be utilized to assess risks and predict market trends. In climate science, HDMMs can facilitate the modeling and prediction of climate change. Healthcare also benefits from HDMMs, enabling the identification of patterns in patient data to enhance diagnostic and treatment approaches. These instances demonstrate HDMMs' diverse applicability across various sectors.
Question 4: What are the limitations of high-dimensional models?
HDMMs, despite their strengths, possess inherent limitations. The complexity of the models themselves can sometimes obscure the interpretability of the results. Accurate interpretation of outcomes requires a deep understanding of the model's underlying mechanics. Over-reliance on complex models without sufficient attention to data quality can potentially lead to erroneous predictions. Furthermore, the computational resources necessary for implementing and maintaining these models can pose significant logistical hurdles.
Question 5: How can the reliability of HDMM predictions be improved?
The reliability of HDMM predictions can be enhanced through several strategies. Robust validation processes are essential to ensure that models accurately reflect real-world systems. Employing sophisticated statistical techniques, ensuring data quality, and meticulously refining the models are all critical factors in improving predictive accuracy and ensuring reliable results. Ongoing monitoring and updates, based on newly available data, further contribute to model refinement and the reliability of predictions.
Understanding these key aspects of HDMMs will aid in effectively interpreting and utilizing these sophisticated models in various applications.
This concludes the FAQ section. The following section will delve into specific applications of HDMMs across various domains.
Conclusion
High-dimensional models (HDMMs) represent a powerful analytical framework for understanding intricate systems. This exploration has highlighted the crucial role of HDMMs in diverse fields, emphasizing their capacity to analyze complex datasets and extract meaningful patterns. Key aspects discussed include the inherent complexity of many real-world phenomena, the importance of robust data analysis techniques, the utility of pattern recognition in predictive modeling, and the iterative process of model refinement. The interdisciplinary nature of HDMM applications was also underscored, demonstrating their applicability across diverse scientific and practical domains. The exploration also touched upon the challenges inherent in implementing and interpreting HDMMs, underscoring the importance of rigorous validation and interpretation methods. Ultimately, HDMMs present a valuable set of tools for handling intricate relationships and forecasting future outcomes.
Moving forward, the advancement and refinement of HDMMs will remain a critical area of focus. Further research and development are necessary to address the limitations associated with handling massive datasets, improving model interpretability, and expanding the range of complex systems amenable to HDMM analysis. As computational power and data availability continue to grow, HDMMs will likely play an increasingly significant role in tackling complex global challenges. Their ability to extract insights from vast amounts of information holds the potential to drive innovation and progress across a wide spectrum of fields.
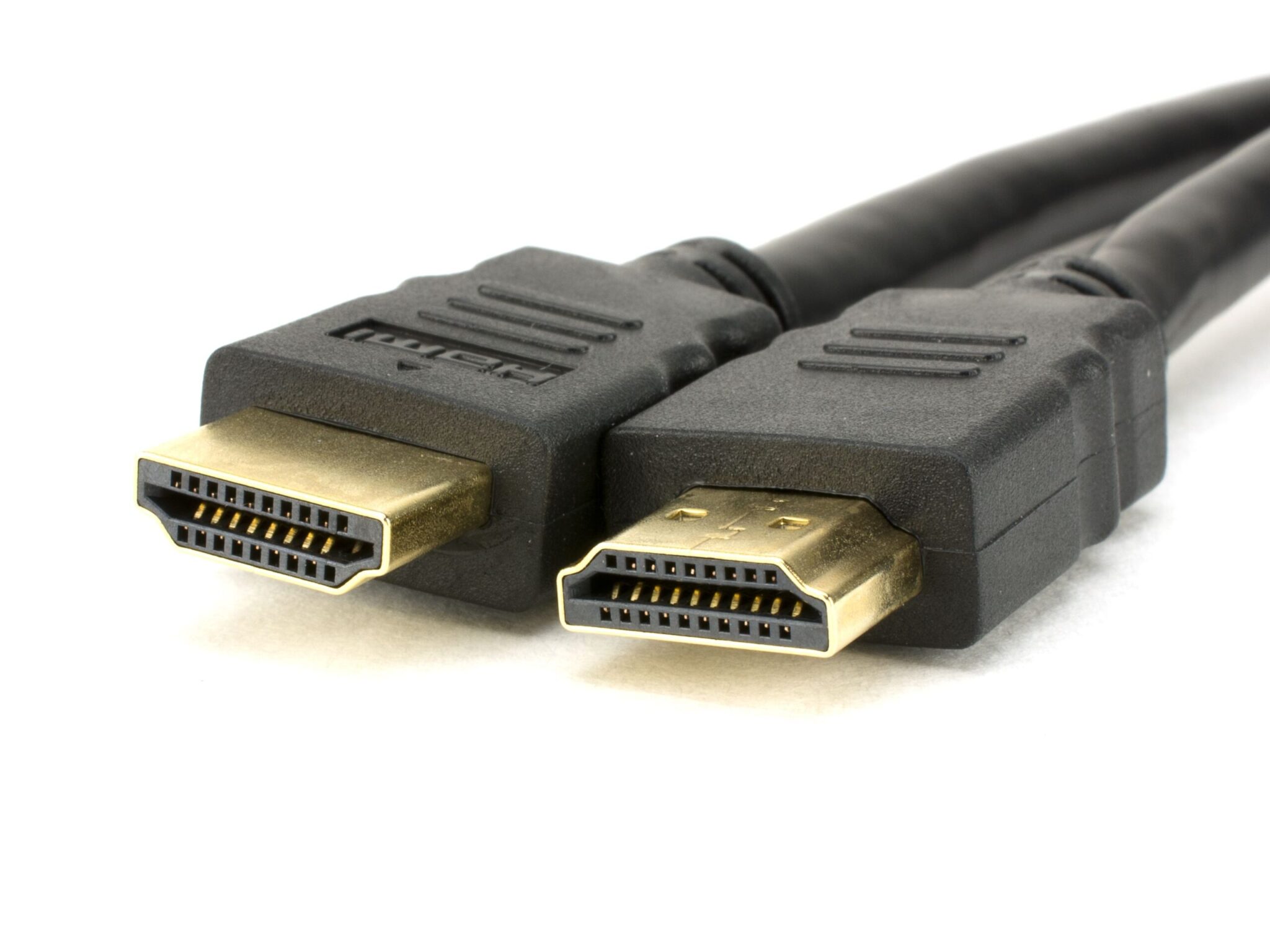

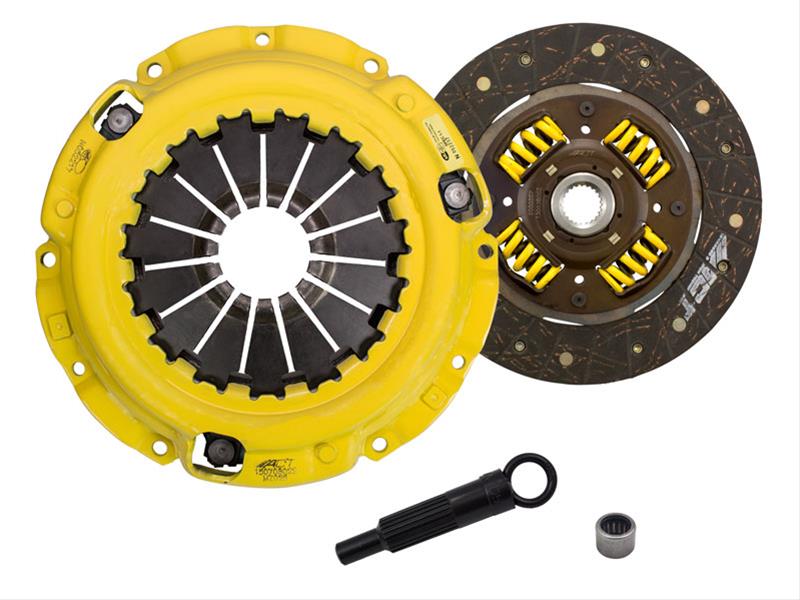
Detail Author:
- Name : Dr. Yoshiko Wiza
- Username : graham.myles
- Email : sanford.palma@sauer.com
- Birthdate : 1989-08-30
- Address : 8082 Rosina Wall Suite 829 Kemmerberg, AL 09785
- Phone : +13603628760
- Company : Nader-Howe
- Job : Paste-Up Worker
- Bio : In sit id ut consequatur aut deserunt porro. Aut molestiae aut ab est. Tempore molestiae necessitatibus tempore quos omnis.
Socials
tiktok:
- url : https://tiktok.com/@raul.kiehn
- username : raul.kiehn
- bio : Recusandae sint commodi dolore dolore atque.
- followers : 381
- following : 1731
instagram:
- url : https://instagram.com/raul_kiehn
- username : raul_kiehn
- bio : Qui magni velit consequatur nemo. Id dignissimos rerum velit nihil soluta.
- followers : 6922
- following : 537
twitter:
- url : https://twitter.com/rkiehn
- username : rkiehn
- bio : Quas autem et qui vel id quae. Est modi tenetur sed consectetur. Iure ut atque quis omnis fugiat.
- followers : 6643
- following : 30
linkedin:
- url : https://linkedin.com/in/raul_dev
- username : raul_dev
- bio : Voluptate exercitationem nihil velit odit.
- followers : 730
- following : 442
facebook:
- url : https://facebook.com/rkiehn
- username : rkiehn
- bio : Quam error voluptatem quia cupiditate et molestiae dolorum.
- followers : 6290
- following : 1532