What is a system for precise measurement and analysis? A tool for quantifying data accurately.
A system for precise measurement and analysis, often used in scientific research and engineering applications, allows for the accurate quantification of data. This process involves the precise measurement of physical attributes and their representation in numerical form. For instance, a system for measuring the acceleration of a particle in a controlled environment would provide precise numerical values for different parameters, like time and distance, to determine acceleration. The data gathered can then be analyzed statistically, allowing for trends and relationships between variables to be discerned.
Such a system is crucial for achieving reliable results in various fields. Its ability to quantify data accurately minimizes error, increasing the validity and reproducibility of experiments and research. The standardization and precision inherent in this approach promote the advancement of scientific knowledge across disciplines. This systematic approach also underpins engineering designs, ensuring products function reliably and efficiently. By providing a quantitative understanding of complex phenomena, the system enhances the overall efficiency and effectiveness of the process.
- Epic Loser Strips Hilarious Fails Funny Fails
- Adorable Pocket Waifus Cute Characters For Your Collection
Moving forward, further exploration into the methodology of measurement will reveal the intricacies of data analysis and its impact across various fields. Understanding the precise techniques and tools utilized in data analysis is vital to comprehend the importance of these measurement systems.
Quantifiable
Accurate measurement and analysis are fundamental to understanding and advancing various fields. Quantifiable aspects are essential for reliable data interpretation and informed decision-making.
- Precision
- Measurement
- Analysis
- Numerical Representation
- Data Interpretation
- Statistical Methods
- Reliability
- Reproducibility
Precise measurement is crucial for accurate analysis. Numerical representation of data allows for application of statistical methods for deeper interpretation. For instance, in physics, quantifying force, acceleration, and distance allows for the formulation of laws. In medicine, analyzing patient data numerically allows for more reliable diagnoses. Reproducibility and reliability in findings are enhanced by focusing on quantifiable data. Understanding these fundamental aspects of quantification is crucial for advancements in various disciplines, from scientific discovery to technological innovation.
1. Precision
Precision is a fundamental component of any robust measurement system. In the context of systems designed for precise measurement and analysis (often referred to in this discussion as a quantification system), high precision is paramount. Without meticulous attention to detail in measurement procedures, the accuracy of subsequent analysis is compromised. For example, in manufacturing, precise measurements of component dimensions ensure parts fit together correctly. In pharmaceutical research, precise measurements of active ingredient concentration are critical for efficacy and safety. These examples underscore the direct correlation between precision and the reliability of results.
The significance of precision extends beyond specific applications. High precision enables the identification of subtle trends and patterns in data, facilitating a deeper understanding of complex phenomena. Precision in measurement minimizes error and allows for the identification of outliers. This refined understanding is invaluable in fields ranging from astronomy, where minute variations in celestial body movements are crucial, to environmental science, where precise measurements of pollution levels inform policy decisions. Furthermore, the accumulation of precise data over time allows for the development of more sophisticated predictive models, improving decision-making and forecasting. Without precision, the accumulation of knowledge would be significantly hampered.
In summary, precision is not simply a desirable characteristic but a necessity within quantification systems. High precision ensures the validity and reliability of results, enabling a deeper understanding of underlying phenomena. The benefits extend to numerous disciplines, fostering advancements in research, manufacturing, and policy-making. Therefore, the development and maintenance of precise measurement methodologies are critical to the advancement of knowledge and progress in various fields.
2. Measurement
Measurement forms the bedrock of quantitative analysis. Its accuracy and precision are essential components of any robust system for quantifying data. Effective measurement underpins the reliability and validity of findings in various fields, from scientific research to engineering design. The process of measurement, when meticulously executed, provides the foundation upon which inferences and conclusions are drawn.
- Defining the Target
A crucial initial step in measurement involves clearly defining the specific attribute or phenomenon to be quantified. Ambiguity in this definition can lead to flawed data and ultimately compromised results. For example, accurately measuring "customer satisfaction" requires a precise definition of what constitutes a satisfied customer. This might involve surveying specific aspects of their experience, like ease of use, product quality, and service responsiveness. This careful definition ensures that data collected truly reflects the intended concept and not something else.
- Selecting Appropriate Tools and Methods
Choosing the correct instruments and methodologies is critical. Different metrics require different tools. Measuring length might involve a ruler or laser caliper; measuring temperature, a thermometer. The selection must align with the desired precision and accuracy. For instance, measuring the concentration of a chemical in a solution necessitates specialized laboratory equipment, while surveying public opinion about a political candidate might involve standardized questionnaires.
- Ensuring Accuracy and Precision
The tools used must be calibrated and employed correctly to ensure both accuracy (the closeness of a measurement to the true value) and precision (the consistency of repeated measurements). In scientific research, the use of standardized protocols and meticulous procedural adherence contribute to both accuracy and precision. Error analysis helps identify and mitigate potential sources of inaccuracies.
- Data Recording and Management
Accurate data recording and effective management systems are necessary for analysis. Clear labeling, consistent units, and structured data formats are essential for efficient analysis. Use of data management software, if applicable, ensures clarity and consistency. An example could be tracking sales data in a dedicated database, enabling efficient analysis of trends and patterns.
Ultimately, the rigor and precision involved in measurement directly impact the trustworthiness of any subsequent analysis or conclusions. Robust measurement frameworks are indispensable for building robust and credible quantitative assessments across diverse fields. By meticulously defining the target, selecting appropriate tools, ensuring accuracy, and efficiently managing data, systems for quantifying information yield reliable and insightful results. This, in turn, paves the way for progress in various domains.
3. Analysis
Analysis is an indispensable component of any robust quantification system. It transforms raw data into actionable insights. The quality and rigor of the analysis directly impact the reliability and validity of conclusions derived from quantified information. Without a thorough analytical process, quantitative data remains inert, devoid of meaningful interpretation. This section explores key facets of analysis essential to effective quantification.
- Data Interpretation
Data interpretation involves extracting meaningful information from quantified data. This process necessitates a deep understanding of the underlying phenomena, statistical methods, and the context within which the data was collected. For example, analyzing sales data might reveal seasonal trends or the effectiveness of marketing campaigns. In scientific research, interpreting data related to experimental variables identifies trends and patterns, leading to theoretical advancements. Effective data interpretation is crucial for drawing valid conclusions and informing strategic decisions.
- Statistical Analysis
Statistical analysis provides a structured framework for interpreting quantified data. Techniques such as regression analysis, hypothesis testing, and correlation analysis allow for the identification of relationships and patterns within data sets. In financial analysis, statistical models predict market trends. In social science research, statistical analysis uncovers correlations between social factors and human behavior. The application of appropriate statistical methods enhances the reliability of conclusions derived from data.
- Error Analysis and Validation
Rigorous analysis inherently involves error analysis and validation. Assessing the potential sources of error in measurement and data collection is crucial for evaluating the reliability of results. Validation techniques ascertain the accuracy and credibility of the data. This process ensures that the analysis is based on sound and trustworthy information. For instance, in engineering designs, error analysis helps identify potential structural weaknesses. In scientific research, validation techniques confirm that experimental procedures and results are accurate. This approach minimizes the impact of inaccuracies on conclusions.
- Trend Identification and Prediction
Analysis frequently involves identifying trends and predicting future outcomes. By examining historical data and trends, patterns emerge that can be used to forecast future performance. Financial forecasting utilizes this principle to predict market fluctuations. In climate science, analysis of historical data allows for projections of future environmental conditions. This forward-looking aspect allows for proactive measures and informed decision-making.
In conclusion, the interplay between quantification and analysis is fundamental to achieving meaningful insights. The methods and approaches employed in analysis directly influence the interpretation and reliability of quantitative data. A strong analytical framework ensures the quality and trustworthiness of conclusions drawn, guiding informed decisions in numerous fields. Robust analysis ensures that quantification efforts yield valuable information and contribute effectively to knowledge advancement.
4. Numerical Representation
Numerical representation is a fundamental component of systems designed for precise measurement and analysis. It bridges the gap between observable phenomena and quantifiable data. Without this crucial step, data remains qualitative and lacks the rigor required for meaningful interpretation and application. Numerical representation allows for the systematic and standardized comparison, analysis, and modeling of diverse phenomena.
The transformation of observations into numerical values is essential to extract insights. Consider scientific experimentation: measuring the height of plants over time requires converting this observation into numerical values (e.g., centimeters). This allows for plotting graphs, calculating growth rates, and determining relationships between variables. Similarly, in manufacturing, precise numerical representations of dimensions guarantee the compatibility of parts. In finance, numerical representation of market data enables sophisticated analysis, enabling the identification of trends and patterns that inform investment decisions. In each example, converting observations into numbers empowers a deeper understanding of the underlying processes.
The significance of numerical representation extends beyond specific applications. By expressing observations numerically, quantification systems can leverage statistical tools, mathematical modeling, and data visualization techniques to uncover hidden relationships and predict future outcomes. This is critical for advancements in various fields, from scientific discoveries to technological innovations. This crucial step facilitates data comparison, statistical analysis, and the development of models that ultimately drive progress in diverse fields. Challenges arise when suitable numerical representations are absent or imprecise, hindering analysis and potentially leading to inaccurate conclusions. Consequently, understanding the principles and methodologies of numerical representation is essential to achieving meaningful results in quantifiable contexts.
5. Data Interpretation
Data interpretation, a critical component of any system designed for precise measurement and analysis, directly impacts the validity and utility of findings. It is the process of extracting meaning from quantified data, transforming raw numbers into actionable insights. Effective interpretation is inseparable from the process of quantificationoften referred to in this context as a "measurement and analysis system." The quality of interpretation hinges on the accuracy and completeness of the data, the appropriateness of the analytical methods employed, and the depth of understanding of the subject matter. In essence, data interpretation is the bridge between raw data and meaningful conclusions.
Consider a manufacturing company analyzing production data. Numerical representations of defect rates, production time, and material costs are quantified. Interpretation of this data reveals trends, identifies bottlenecks, and suggests improvements. If the data interpretation phase is insufficient, the company might miss opportunities to optimize operations, leading to increased costs and reduced efficiency. Similarly, in clinical trials, the numerical representation of patient responses to treatments is crucial. Careful interpretation of these data determines the effectiveness and safety of new medications, directly impacting patient outcomes. A flawed interpretation process could lead to misleading conclusions and jeopardize the development or implementation of potentially life-saving treatments. In both cases, the quality of data interpretation directly influences the practical application and effectiveness of the quantification system.
Effective data interpretation relies on a strong understanding of the principles of measurement and analysis. Challenges can arise when data sets are complex, when the tools for analysis are inadequate, or when sufficient context is lacking. In all cases, accurate and insightful interpretation is essential for deriving valid conclusions from quantified data, leading to informed decisions and impactful advancements in various fields. This emphasis on data interpretation underlines the importance of understanding the underlying phenomena the system is designed to study.
6. Statistical Methods
Statistical methods are indispensable tools within systems for precise measurement and analysis. Their role is to extract meaningful information from quantified data, transforming raw numbers into insights. Statistical methodologies are crucial to establishing reliability, understanding relationships, and drawing valid conclusions from data produced by quantification systems, effectively connecting the quantitative data with a deeper understanding of phenomena.
- Descriptive Statistics
Descriptive statistics summarize and describe collected data. Measures like mean, median, mode, standard deviation, and frequency distributions condense complex datasets into readily understandable summaries. In quality control, descriptive statistics are used to identify production process variations and pinpoint potential issues. In market research, these summaries reveal consumer preferences, aiding informed business decisions.
- Inferential Statistics
Inferential statistics extend beyond description to make inferences about populations based on samples. Methods like hypothesis testing and confidence intervals allow for generalizations about a larger group from a smaller representative subset. This is crucial in medical research, where sample populations are used to understand the efficacy and safety of new treatments. In opinion polling, inferential statistics allow for estimations of public sentiment about political candidates or issues.
- Probability and Distributions
Probability theory provides the mathematical framework for understanding uncertainty and chance variation in data. Probability distributions describe the likelihood of different outcomes. This is critical in forecasting, predicting outcomes (such as weather patterns, stock market fluctuations, or the spread of diseases) based on past data and probabilistic models derived through analysis. The comprehension of probability principles is fundamental to the interpretation of any quantitative data set.
- Correlation and Regression Analysis
These methods identify relationships and dependencies between variables. Correlation analysis measures the strength and direction of a relationship between variables, while regression analysis models the relationship, potentially to forecast values. In economics, understanding relationships between interest rates and inflation, or consumer spending and GDP growth, are achieved via these methods. Similarly, in biological sciences, scientists use these analyses to explore how variables like temperature and humidity affect plant growth.
The application of statistical methods to data obtained from precise measurement systems is essential to move beyond simple description and into meaningful interpretation and prediction. These methods provide the rigor and structure needed to understand and communicate findings in a reliable and insightful manner, ultimately contributing to knowledge advancement across numerous fields. The effectiveness and validity of the quantification process depend crucially on the appropriate application and interpretation of these statistical tools.
7. Reliability
Reliability, in the context of systems designed for precise measurement and analysis (often referred to as quantification systems), is a crucial attribute. The ability of a system to consistently produce accurate and dependable results is paramount for validity and meaningful insights. Without reliability, the data collected from such systems loses its value and potentially leads to erroneous conclusions. This aspect underscores the importance of robust methodologies, standardized procedures, and meticulous quality control in measurement and analysis.
- Consistency and Reproducibility
A reliable quantification system consistently delivers similar results under the same conditions. Reproducibility is equally importantdifferent researchers or instruments, using the same methodology, should achieve comparable results. In scientific experiments, repeated measurements of a physical constant should yield similar values. In manufacturing, reliable instruments consistently measure dimensions, ensuring product quality and conformity. If results are inconsistent, or highly variable, the accuracy and trustworthiness of the entire system are severely compromised.
- Accuracy and Precision
Reliability is interwoven with accuracy and precision. Accurate measurements reflect the true value of the attribute being measured. Precision signifies the consistency of repeated measurements. A reliable system minimizes errors, ensuring both accuracy and precision in the data collected. In engineering, accurate measurement of dimensions ensures proper component fit and function. In medical diagnostics, reliable test results guide accurate diagnoses and treatment plans. Inaccurate or imprecise data is problematic, as it can lead to unreliable conclusions and ineffective interventions.
- Minimization of Bias and Error
A reliable quantification system minimizes sources of bias and error. Bias could arise from systematic flaws in the instruments, methods, or data collection process. Error could be random variations, introducing variability in measurements. Identifying and mitigating these sources are key to reliable results. In surveying, careful methodology and well-designed sampling techniques reduce bias and random error, leading to more reliable conclusions about the target population. In environmental monitoring, consistent calibration of instruments and rigorous data quality control minimize errors and biases that might distort the assessment of environmental conditions.
- Standardization and Calibration
Reliability is often achieved through standardization and calibration. Standardized procedures and instruments ensure consistency and comparability across different measurement instances. Calibration ensures the instruments used are accurate by regularly adjusting them against known standards. This is critical in scientific research and industrial settings to maintain reliability in measurements. In quality control, standardized operating procedures (SOPs) and calibrated equipment guarantee consistent product quality. Without standardization and regular calibration, measurements cannot be relied upon, potentially jeopardizing outcomes in critical areas such as healthcare and engineering.
In conclusion, reliability within a quantification system is not a singular facet but rather a collection of interconnected attributes. Consistency, accuracy, minimization of bias and error, and proper standardization are all intertwined. By focusing on these factors, the validity and trustworthiness of measurements and analyses are greatly enhanced. This ultimately allows for more robust conclusions and informed decision-making across diverse fields. Without reliability, the entire quantification process loses its value and impact.
8. Reproducibility
Reproducibility, a cornerstone of scientific rigor and a critical component of effective measurement systems, is intrinsically linked to the concept of precise quantification. The ability to replicate results, to obtain similar outcomes under identical conditions, underscores the validity and reliability of findings generated by quantitative processes. Without reproducibility, the trustworthiness of data diminishes, hindering advancements in any field relying on quantifiable information.
- Consistency in Measurement Procedures
Reproducibility necessitates consistent and well-defined procedures for data collection. This encompasses the meticulous documentation of experimental methods, instrumentation calibration, and environmental factors. In scientific experiments, identical protocols allow independent researchers to obtain comparable outcomes. In quality control, standardized procedures guarantee the consistency of manufactured products. Deviation from established protocols introduces error and reduces the likelihood of reproducible results.
- Standardization of Instruments and Materials
Standardization of instruments and materials is essential for reproducible outcomes. Calibration of equipment ensures accurate measurements, minimizing variations in data collection. Using identical materials and reagents in experiments further reduces potential sources of variability. In medical diagnostics, standardized test procedures and calibration of instruments ensure reliable and comparable results across different laboratories. Inaccurate calibration or the use of non-standardized materials directly compromises the reproducibility of measurements.
- Control of External Variables
Reproducible results demand rigorous control over external variables that could influence the outcome. In scientific investigations, careful monitoring and control of factors like temperature, pressure, and light intensity are necessary. In industrial settings, controlling factors such as humidity or specific chemical compositions is crucial to ensuring consistent product quality. External variable fluctuations introduce uncertainties that undermine reproducibility.
- Data Integrity and Analysis
Reproducible results extend to data analysis. Uniform protocols for data handling, statistical analysis, and report writing ensure that others can understand and replicate the methodologies. Ensuring data accuracy and transparency in the analysis process fosters reproducibility. In large-scale studies, the use of dedicated data management systems and rigorous auditing procedures helps preserve data integrity and contributes to reproducibility of findings.
In conclusion, reproducibility is integral to any robust system of precise quantification. Standardized procedures, calibrated instruments, controlled environments, and transparent analytical practices are indispensable for achieving trustworthy and replicable results. This, in turn, strengthens the validity and credibility of findings, allowing for the accumulation of knowledge and the advancement of understanding in various fields.
Frequently Asked Questions (FAQ) Regarding Precise Measurement and Analysis Systems
This section addresses common questions and concerns regarding precise measurement and analysis systems. These systems, crucial in numerous fields, are characterized by accuracy, precision, and reliability in data collection and interpretation.
Question 1: What are the essential components of a robust measurement and analysis system?
A robust system requires precise measurement tools, standardized procedures, and reliable data analysis methods. Accurate instrumentation, calibrated to established standards, is fundamental. Well-defined procedures for data collection and analysis minimize error and ensure consistency. Sophisticated statistical methods are essential for extracting meaningful insights from the quantified data.
Question 2: How does precision affect the validity of results?
High precision in measurement is directly linked to the validity of results. Precise measurements minimize errors, leading to more accurate representations of the target attribute or phenomenon. Inaccurate or imprecise measurements introduce variability and uncertainty, compromising the reliability of any subsequent analysis and conclusions.
Question 3: What role do statistical methods play in data interpretation?
Statistical methods are essential for interpreting data from quantification systems. Descriptive statistics summarize the data, while inferential statistics enable drawing conclusions about larger populations based on samples. Statistical techniques such as correlation and regression analysis provide insight into relationships between variables, facilitating predictive modeling and informed decision-making.
Question 4: How can bias and error be minimized in data collection and analysis?
Minimizing bias and error requires careful consideration at every stage, from instrument calibration to data analysis. Standardization of procedures, meticulous control of external variables, and appropriate error analysis techniques are crucial for mitigating potential sources of bias. Data validation steps help identify and correct errors, contributing to trustworthy results.
Question 5: What is the importance of reproducibility in measurement and analysis?
Reproducibility is vital for the reliability and credibility of findings. The ability to replicate results under identical conditions demonstrates the robustness of the measurement and analysis system. Reproducible outcomes are essential in scientific research, quality control, and other fields where consistent results are critical for informed decision-making.
Understanding these FAQs provides insight into the critical elements of reliable and precise measurement and analysis systems. By adhering to best practices in instrumentation, methodology, and analysis, the trustworthiness and utility of these systems are maximized.
Moving forward, exploring the practical applications of these systems in various industries and research disciplines is crucial.
Conclusion
This exploration of precise measurement and analysis systems, often central to fields as diverse as scientific research and industrial production, reveals the fundamental importance of quantifiable data. The article emphasizes the interconnectedness of accurate measurement, rigorous analysis, and reliable interpretation. Key components like precision, reproducibility, and the appropriate application of statistical methods emerge as crucial for the validity and utility of findings. The meticulous documentation of methodologies and the consistent application of standards are vital to ensuring the trustworthiness of the results. The necessity for accurate data representation, error analysis, and thorough validation procedures in the context of data interpretation is underscored throughout. Ultimately, the article highlights that robust systems for precise measurement and analysis provide a pathway for advancing knowledge and driving progress across various disciplines.
The consistent and accurate quantification of phenomena, whether in laboratories or industrial settings, underpins informed decision-making, facilitates technological advancement, and drives a deeper understanding of the world around us. The significance of precise measurement and analysis underscores the imperative for continued development and refinement of methodologies to ensure ever-increasing reliability and validity in data collection and interpretation. Future progress in these systems promises further breakthroughs in numerous fields, pushing the boundaries of knowledge and innovation.
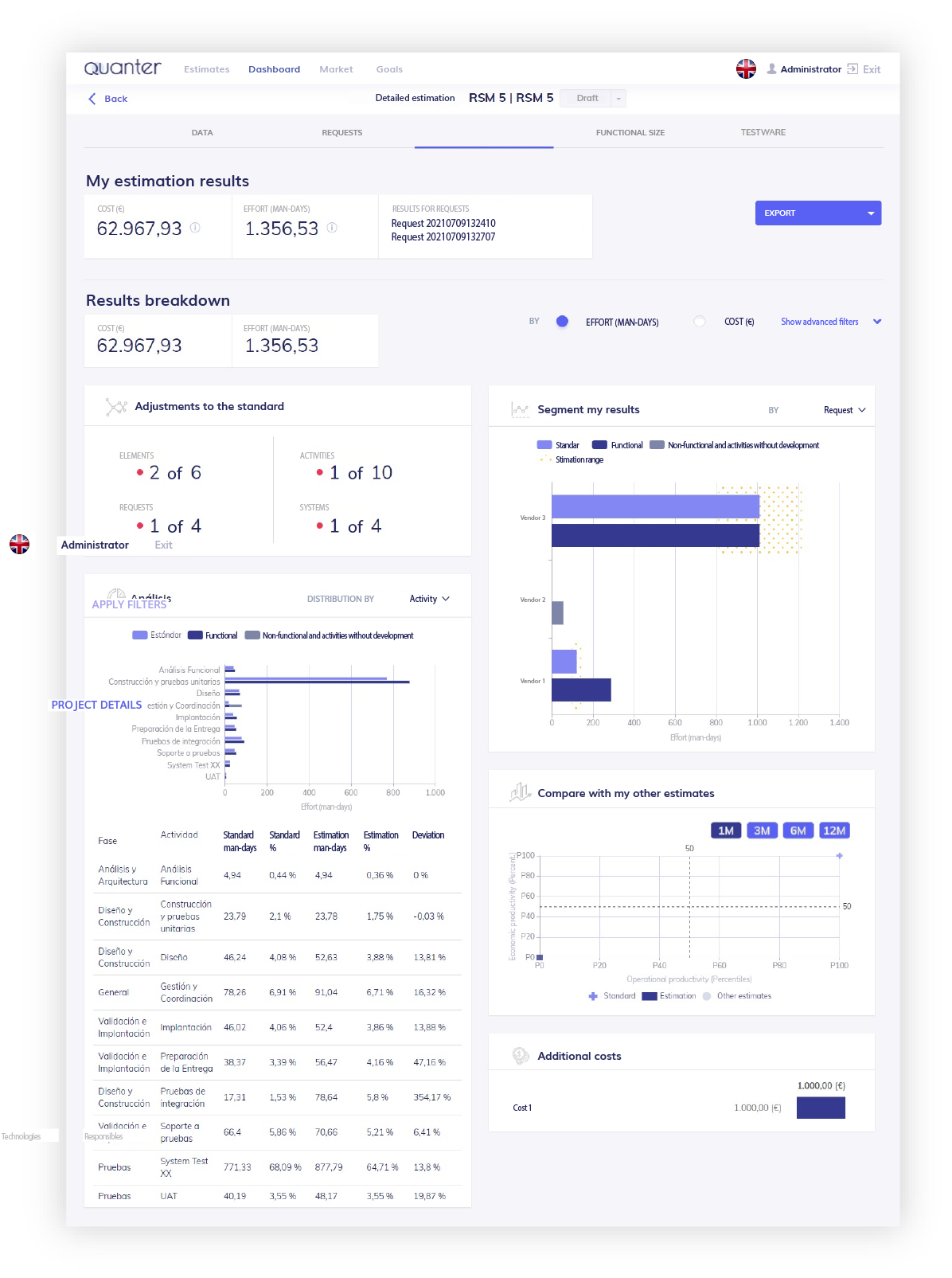
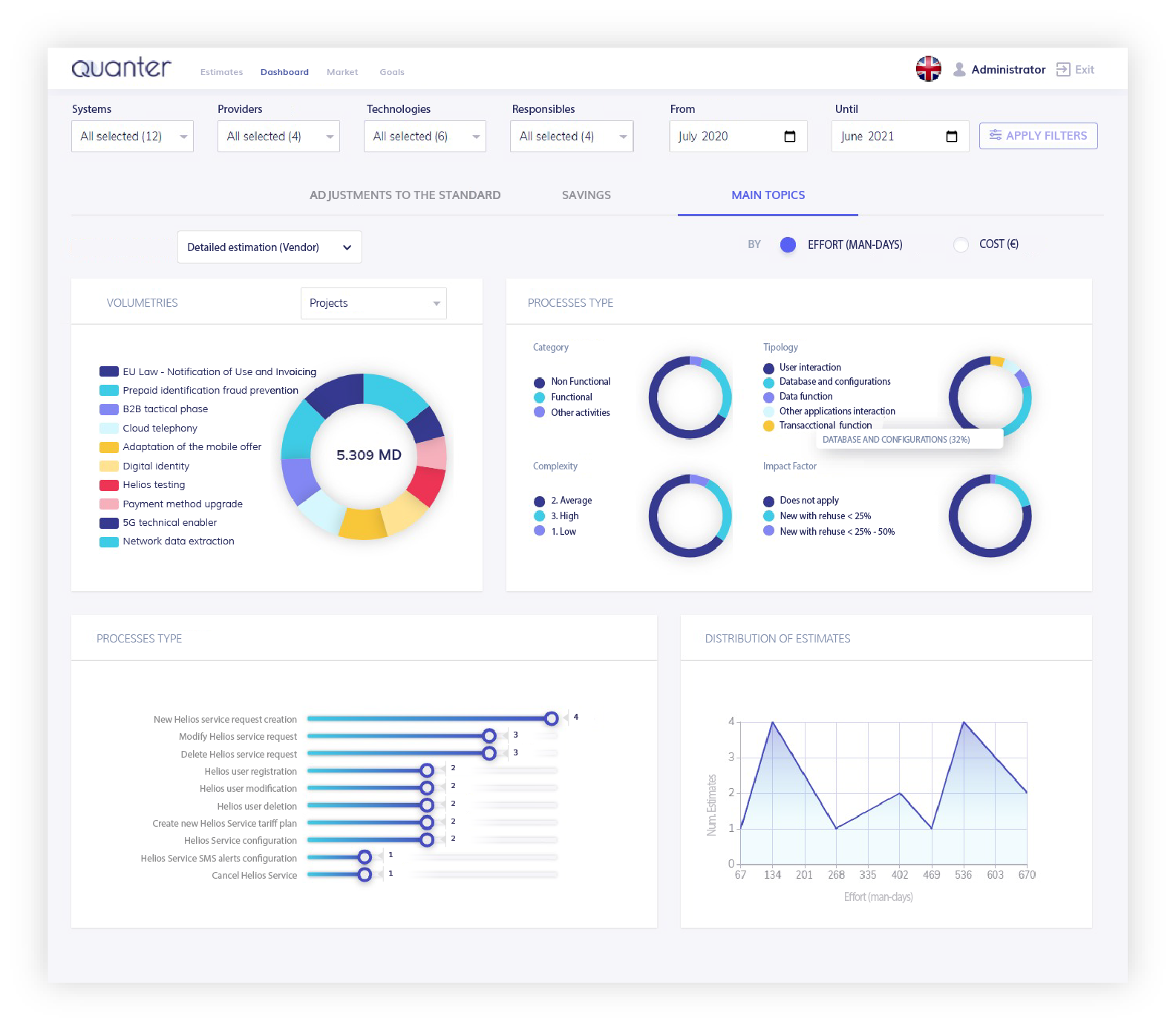

Detail Author:
- Name : Mossie Wiegand
- Username : gschmidt
- Email : jacobson.isai@feil.biz
- Birthdate : 1973-10-24
- Address : 141 Tessie Orchard Nicoburgh, GA 78613-3853
- Phone : (484) 605-4105
- Company : Walsh, Wilkinson and Wolff
- Job : Bartender
- Bio : Ut quaerat blanditiis quia quia non officiis. Consequatur cupiditate sed perspiciatis enim beatae. Non dolores unde vero est aliquam.
Socials
twitter:
- url : https://twitter.com/sister_brown
- username : sister_brown
- bio : Labore quam omnis qui saepe dolorem natus quidem. Autem earum quos at ut rerum eum. Aut et fugit sed voluptas.
- followers : 5041
- following : 223
facebook:
- url : https://facebook.com/brown2013
- username : brown2013
- bio : Est nulla quaerat molestiae autem porro.
- followers : 5193
- following : 2318
instagram:
- url : https://instagram.com/sister_brown
- username : sister_brown
- bio : Enim quis placeat fuga. Officiis labore dolor in voluptatem sit dicta nemo.
- followers : 5981
- following : 2878
tiktok:
- url : https://tiktok.com/@brown1986
- username : brown1986
- bio : Et ea et eos explicabo. Sequi et et iusto reprehenderit eligendi facilis.
- followers : 3803
- following : 2861