What is the significance of this specialized computational approach? A groundbreaking methodology is revolutionizing complex simulations.
This approach, a specialized computational method, offers a powerful framework for modeling intricate systems. It facilitates detailed analyses by integrating diverse data sources and employing advanced algorithms. By leveraging this technique, researchers can dissect complex interactions and obtain insights unattainable through traditional methods. Examples include scenarios across various fields, like biological processes, engineering designs, or financial modeling, where the interactions are multifaceted.
The benefits of this approach lie in its potential to accelerate scientific discovery and technological advancement. By providing accurate and detailed predictions, this method fosters informed decision-making in various sectors. Furthermore, it enables researchers to understand previously uncharted territories, ultimately accelerating progress in fields ranging from materials science to astrophysics. Its importance stems from the potential to model extremely complex systems, generating crucial data insights for advancements in various fields. The method, by its inherent design, surpasses the limitations of older methodologies and brings substantial advances in efficiency, reliability, and predictability.
Now, let's explore the practical applications of this innovative approach in detail.
cemvx
Understanding the core components of cemvx is crucial for appreciating its multifaceted applications. This approach to complex systems modeling provides a sophisticated framework for analysis.
- Computational
- Modeling
- Integration
- Analysis
- Prediction
- Optimization
These key aspects, from computational modeling to optimization, highlight cemvx's comprehensive nature. Computational methods form the bedrock, enabling sophisticated modeling of intricate systems. Data integration allows for a holistic view, while analysis unveils key patterns and relationships. Accurate prediction empowers informed decision-making, and optimization seeks to enhance system performance. For instance, in financial modeling, cemvx can simulate market fluctuations with sophisticated integration of various factors, leading to improved forecasting and investment strategies. Similarly, in materials science, cemvx can aid in designing new materials with optimized properties by simulating atomic interactions and predicting macroscopic behavior. This detailed approach thus offers profound insights across various disciplines.
1. Computational
Computational methods underpin the core functionality of cemvx. Their role in this specialized approach is paramount, driving the intricate modeling and analysis of complex systems. This section delves into the crucial aspects of computation within the cemvx framework, highlighting the essential techniques and their practical application.
- Algorithm Design and Implementation
The effectiveness of cemvx hinges on the sophistication of the algorithms employed. These algorithms meticulously translate theoretical concepts into practical computational procedures. Examples encompass intricate numerical methods for solving differential equations, optimization routines for finding optimal solutions, and sophisticated statistical techniques for interpreting data. The success of the cemvx approach relies heavily on the efficiency and accuracy of these implementations.
- Data Representation and Manipulation
The ability to represent and manipulate complex data accurately is essential for cemvx. This involves converting real-world phenomena into numerical formats, processing vast datasets, and performing transformations to derive meaningful insights. Methods like discretization, parameterization, and standardization play a critical role in preparing data for computational analysis. For instance, in simulating biological systems, representing protein structures and interactions computationally is vital for predicting behavior.
- Model Validation and Verification
Rigorous verification and validation processes are integral components of cemvx. This involves evaluating the accuracy and reliability of computational models against empirical data or known theoretical results. Techniques for validation often include comparing computational outcomes with experimental results, sensitivity analyses, and assessing model assumptions. Effective validation minimizes potential errors and enhances the confidence in cemvx predictions.
- Scalability and Efficiency
The ability to handle large datasets and intricate simulations is vital for cemvx's application to complex problems. Efficient computational resources and optimized algorithms are crucial to ensure the method's applicability to real-world scenarios. Advanced techniques such as parallel computing and high-performance computing are often integral to the efficient execution of cemvx simulations.
In essence, the computational aspects of cemvx are not merely supplementary tools but rather the fundamental building blocks of the approach itself. The innovative techniques and methodologies employed drive the analytical power and predictive capabilities of cemvx, ultimately leading to a more thorough understanding of intricate systems across diverse disciplines.
2. Modeling
Modeling plays a central role in cemvx. It represents the core process of translating complex systems into simplified, computational representations. This simplification allows for the exploration of system behavior, the identification of key interactions, and the prediction of outcomes under various conditions. The accuracy and fidelity of the model directly influence the reliability of insights derived from cemvx.
- System Abstraction
Modeling involves abstracting a complex system, focusing on essential components and interactions while disregarding irrelevant details. This simplification reduces computational demands and enhances analytical tractability. For instance, in simulating a biological pathway, the model might focus on key enzymatic reactions, neglecting the precise configurations of individual molecules within the cell. This abstraction allows for a more general understanding of the pathway's overall dynamics.
- Parameterization and Input Specification
A crucial aspect of modeling in cemvx is the accurate definition of parameters and inputs. These inputs reflect the specific conditions of the system under investigation. For example, in a financial model, input parameters might include interest rates, market volatility, and investor behavior. The precision of these parameters significantly influences the output's reliability. Incorrect input parameters can lead to inaccurate predictions.
- Behavioral Representation
Models in cemvx aim to capture the essential behavior of the system. This encompasses outlining the dynamic relationships between components and defining how these interactions evolve over time. A model might describe how the concentration of reactants changes during a chemical reaction. Proper representation of behavior is crucial for simulating the system's responses to external stimuli or internal changes.
- Validation and Refinement
The accuracy and reliability of models in cemvx are essential. Validation involves comparing model predictions to empirical data or known theoretical results. If discrepancies emerge, the model must be refined to improve its accuracy. This iterative process of validation and refinement is vital for the continued development and application of cemvx methodologies.
In summary, modeling within the cemvx framework is not just a technical procedure; it's the fundamental lens through which complex systems are analyzed. The chosen models, their parameters, and the fidelity of their representations directly affect the validity of insights generated by cemvx. Careful attention to these aspects is critical to extract useful and reliable conclusions from cemvx's computational approach.
3. Integration
Integration is a critical component of cemvx, enabling the seamless combination of diverse data sources and methodologies within the modeling framework. Its importance lies in the ability to capture the complexity inherent in real-world systems by encompassing multiple interacting factors. This holistic approach allows for a more accurate representation of the system and facilitates more nuanced predictions and analyses.
- Data Fusion from Multiple Sources
Cemvx often requires data from diverse sources, each with its own structure and limitations. Integration allows for the fusion of this disparate data into a unified format usable within the computational model. For example, in environmental modeling, integration might involve combining meteorological data, hydrological data, and land-use information to simulate ecosystem dynamics. This approach enhances the realism of the model, incorporating a broader spectrum of influences on the system being studied.
- Combining Different Modeling Techniques
Cemvx may draw on various modeling approaches, each providing unique insights into specific aspects of the system. Integration facilitates the combination of these methods, allowing for a more comprehensive understanding of the overall system behavior. For instance, in epidemiological modeling, integrating agent-based models with statistical models can provide a more complete picture of disease transmission patterns, accounting for individual-level interactions while simultaneously leveraging the predictive power of statistical analyses.
- Interfacing with Experimental Data
Cemvx often relies on experimental data to validate and calibrate models. Integration ensures the seamless connection between computational models and experimental findings, allowing for the comparison of theoretical predictions with observations from the real world. In materials science, for instance, integration allows incorporation of experimental data on material properties to refine and validate the computational models of the material's behavior under different conditions.
- Handling System Complexity
Integration is essential for tackling the complex interactions inherent in multifaceted systems. By combining diverse data and modeling techniques, cemvx can address the interdependencies and intricate relationships within the system. This is especially relevant in systems like financial markets, where the interplay between various factors (e.g., interest rates, investor sentiment, macroeconomic conditions) necessitates an integrated approach for accurate predictions.
The multifaceted nature of cemvx necessitates a robust integration strategy. This strategy ensures that the model accurately reflects the intricate relationships within the system, enabling comprehensive analyses and reliable predictions. By effectively connecting various data sources and modeling approaches, cemvx can provide a more holistic understanding of the system under study.
4. Analysis
Analysis is integral to the cemvx approach. The core strength of cemvx lies in its ability to extract meaningful insights from complex simulations. Analysis serves as the critical bridge between raw data generated by the computational models and the actionable knowledge gleaned from them. Without rigorous analysis, the output of cemvx simulations remains largely inert, lacking the capacity to inform decision-making or advance understanding of the systems being modeled. This critical connection between analysis and cemvx hinges on the identification of patterns, trends, and relationships within the data, translating the computational results into actionable knowledge.
Real-world applications illustrate this interplay. In financial modeling, cemvx might simulate market fluctuations. Analysis then identifies correlations between different asset classes or the impact of macroeconomic factors on market trends, revealing opportunities or potential risks. In climate modeling, cemvx could simulate future scenarios. Analysis, in turn, pinpoints critical thresholds for environmental change and helps project consequences, enabling informed strategies for mitigation. In drug discovery, cemvx might model protein-ligand interactions. Analysis of these simulations uncovers potential drug candidates, enabling targeted development of novel therapies. Consequently, the power of cemvx directly depends on the analytical techniques applied to the outputs of the computational simulations, translating complex data into actionable information.
In essence, analysis within the context of cemvx is not a separate step but an intrinsic element of the modeling process. It demands sophisticated techniques, ranging from statistical methods to machine learning algorithms, to extract meaningful patterns and make reliable predictions. The robustness and effectiveness of cemvx depend on the thoroughness and sophistication of these analytical methods. Challenges include managing the volume of data generated by complex simulations and ensuring the reliability of the insights derived from the analysis. Overcoming these hurdles will further enhance the predictive capabilities and practical application of cemvx across various domains.
5. Prediction
Prediction, a crucial element of cemvx, plays a pivotal role in harnessing the power of complex simulations for practical applications. Accurate prediction is paramount for anticipating future scenarios and enabling proactive decision-making across diverse fields. This exploration examines the multifaceted role of prediction within the cemvx framework.
- Model Validation and Refinement
Prediction is integral to validating the accuracy of cemvx models. By comparing predicted outcomes with observed data, the reliability and validity of the model can be assessed. Discrepancies between prediction and reality necessitate model refinement, leading to improved accuracy and enhanced predictive power. For instance, in meteorological forecasting, cemvx models predict weather patterns. Validation involves comparing predictions with actual weather data, identifying model weaknesses and leading to more precise future predictions.
- Scenario Analysis and Risk Assessment
Cemvx's predictive capabilities allow for the exploration of various potential future scenarios. By simulating different conditions and inputs, models can project potential outcomes, facilitating risk assessment and strategic planning. In financial modeling, cemvx can predict market fluctuations under different economic conditions, enabling investors to understand potential risks and rewards more thoroughly. The ability to anticipate possible outcomes empowers proactive risk mitigation.
- Trend Identification and Pattern Recognition
Cemvx can identify long-term trends and underlying patterns within data, allowing for anticipatory action. By analyzing data across various conditions, models can unveil potential shifts or evolutions in the system's behavior. In epidemiological modeling, cemvx predicts disease outbreaks by identifying trends in infection rates and contact patterns, allowing for timely public health interventions.
- Optimization and Decision Support
Prediction enables cemvx to drive optimization and support decision-making. By modeling future outcomes under various conditions, cemvx can identify optimal strategies. In supply chain management, cemvx can predict demand fluctuations, allowing companies to optimize their inventory levels and resource allocation, minimizing costs and maximizing efficiency. This optimized approach leverages predictive insights for improved decision support.
In conclusion, prediction is not a standalone element but an essential component of cemvx, deeply intertwined with validation, scenario analysis, pattern recognition, and decision support. The predictive power of cemvx, by leveraging these interconnected components, empowers decision-making in numerous sectors and leads to a more profound understanding of intricate systems.
6. Optimization
Optimization is a critical component of the cemvx framework, fundamentally impacting its application and outcomes. The ability to identify and implement optimal solutions within complex systems is a direct consequence of cemvx's modeling and analytical capabilities. This optimization process, enabled by cemvx, seeks to maximize desired outcomes or minimize undesirable effects based on the specific context of the system being studied. Real-world applications underscore the practical significance of this aspect, illustrating how cemvx drives efficiency and effectiveness in diverse fields.
Consider, for example, a manufacturing process. Cemvx can model the interactions between various production stages, encompassing material flow, energy consumption, and labor allocation. Through analysis of this model, optimal configurations for resource allocation can be determined, potentially leading to a significant reduction in production costs. Similarly, in financial markets, cemvx can simulate market dynamics, including factors such as volatility and investor behavior. By analyzing these simulations, optimal investment strategies can be identified, minimizing risk and maximizing returns. This optimization capability isn't restricted to these examples but extends across various sectors like transportation logistics, energy management, and pharmaceutical development.
The integration of optimization with cemvx significantly enhances the predictive capabilities and practical utility of complex system modeling. By identifying optimal parameters and strategies, cemvx empowers informed decision-making. However, challenges remain in accurately defining objective functions and handling the computational complexity inherent in some optimization problems. Further research is needed to address these challenges and improve the scope and applicability of cemvx within optimization contexts. The continuous advancement of computational capabilities, combined with refined analytical methodologies, will likely further expand the role of optimization within the broader framework of cemvx, thereby enhancing its utility in problem-solving across multiple disciplines.
Frequently Asked Questions (cemvx)
This section addresses common inquiries regarding the cemvx approach to complex systems modeling. Answers are provided in a concise and informative manner.
Question 1: What is the core function of cemvx?
Cemvx serves as a framework for modeling and analyzing complex systems. It integrates diverse data sources and computational methods to generate insights and predictions regarding system behavior. This approach aims to address the complexities often encountered in real-world systems, enabling a deeper understanding of their interactions and outcomes.
Question 2: How does cemvx differ from traditional modeling methods?
Cemvx distinguishes itself through its integrated approach. Unlike traditional methods that might focus on isolated components, cemvx considers the intricate interplay of various factors within a system. This holistic perspective allows for more accurate predictions and analyses, particularly in complex systems where interactions are crucial.
Question 3: What types of data can cemvx handle?
Cemvx can accommodate diverse data types, including numerical, categorical, and experimental data. The ability to integrate and analyze data from various sources, both quantitative and qualitative, is a key aspect of the framework's applicability across different domains.
Question 4: What are the limitations of the cemvx approach?
While powerful, cemvx has limitations. The complexity of some systems might overwhelm the computational resources required for analysis. Furthermore, the accuracy of predictions relies heavily on the quality and completeness of input data. The sophistication of cemvx necessitates expertise in both data analysis and computational modeling.
Question 5: What are some practical applications of cemvx?
Cemvx finds applications across various fields, from financial modeling and engineering design to environmental science and public health. Its ability to simulate and analyze complex systems enables more informed decision-making and contributes to advancing knowledge in diverse disciplines.
In summary, cemvx provides a robust framework for modeling intricate systems. Understanding its core principles and limitations is crucial for effective application.
This concludes the FAQ section. The next section will explore the practical implementations of cemvx within various industries.
Conclusion
The cemvx approach to complex systems modeling offers a powerful methodology for understanding and predicting intricate behaviors. This article has explored the key components of cemvx, including its computational foundations, modeling techniques, data integration strategies, analytical methodologies, predictive capabilities, and optimization potential. The framework's ability to handle diverse data types and methodologies within a unified framework underscores its versatility across a wide range of disciplines. Key strengths include the facilitation of accurate predictions, detailed scenario analysis, and the identification of optimal solutions within complex systems. The framework's inherent adaptability, allowing for integration of various data sources and modeling approaches, highlights its potential to address increasingly complex challenges in modern research and industry.
Moving forward, continued refinement of cemvx methodologies, coupled with advancements in computational power, promises to further enhance its predictive accuracy and practical application. The framework's capacity to integrate diverse data sources and model complex interactions suggests a significant role in driving future discoveries and technological advancements. Researchers and practitioners in diverse fields should consider cemvx as a potential tool for tackling complex problems and unlocking new levels of understanding in their respective domains.
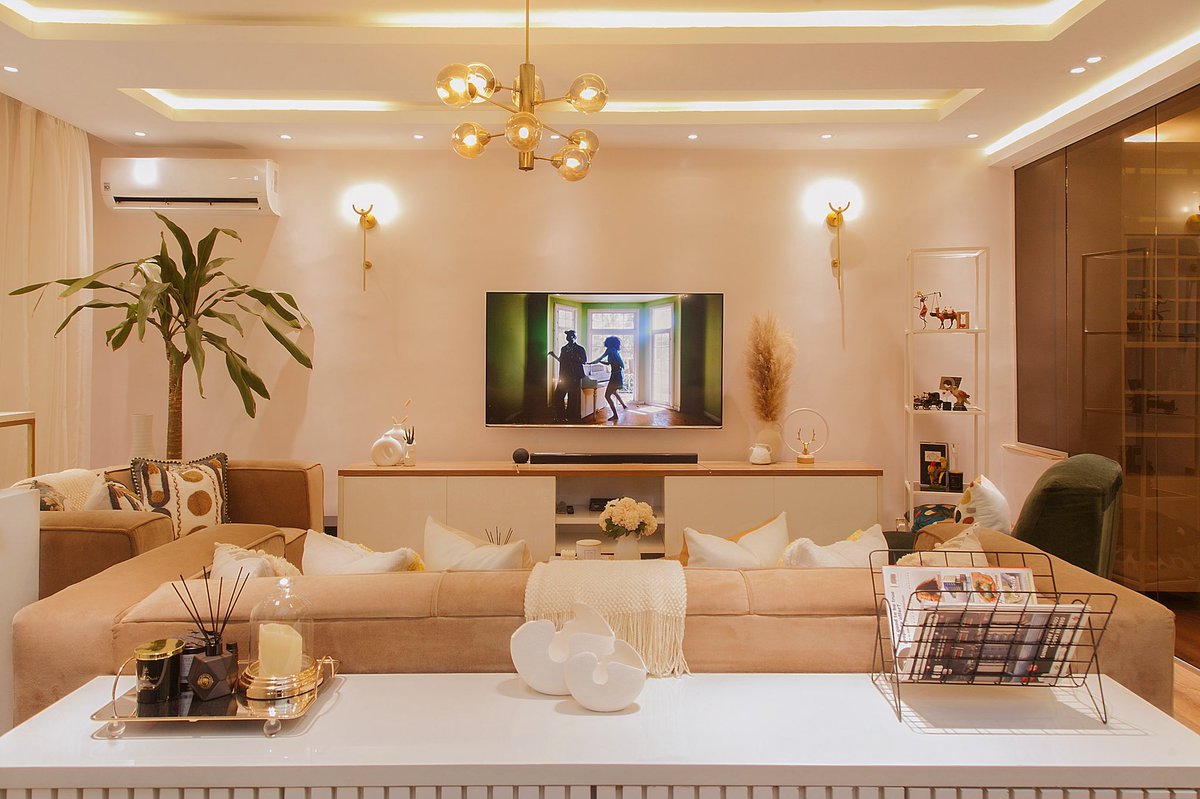
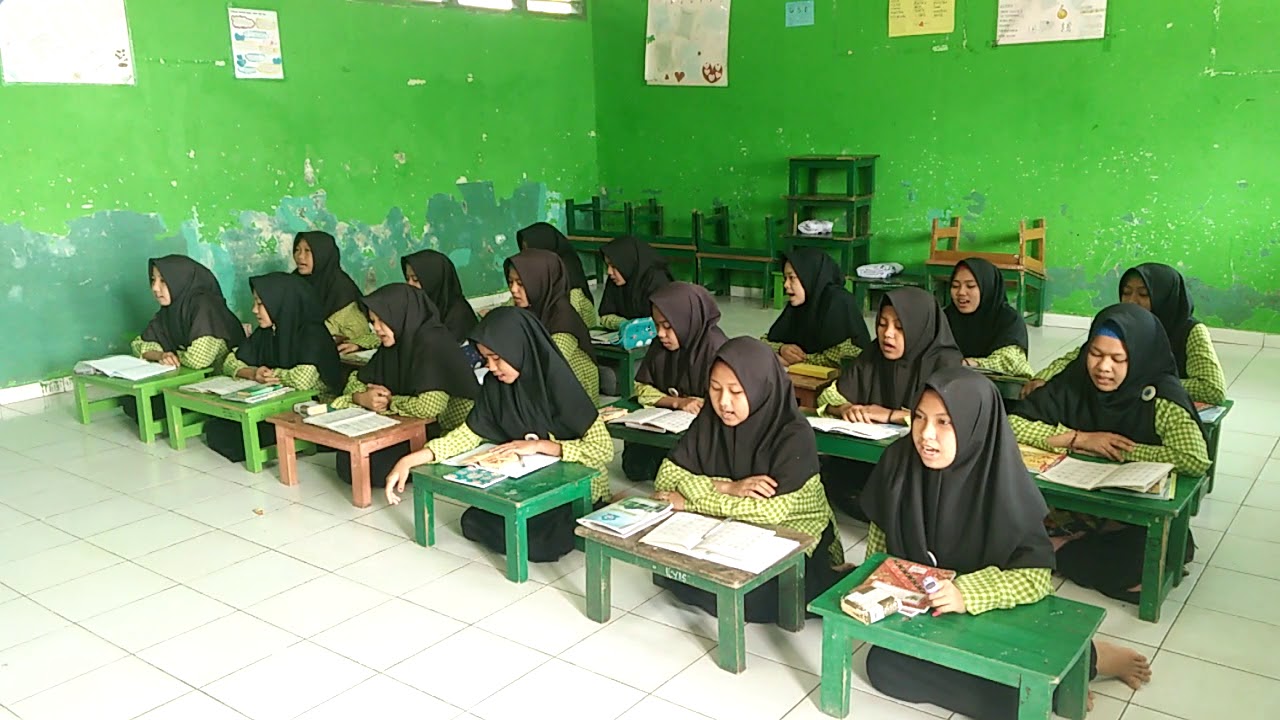
Detail Author:
- Name : Kevon Wisoky
- Username : janie30
- Email : delia.walter@dicki.com
- Birthdate : 1986-04-12
- Address : 370 Palma Landing Suite 444 Schultzland, FL 15558
- Phone : +1-947-689-6675
- Company : Bernier, Crona and Lubowitz
- Job : Production Worker
- Bio : Doloribus voluptatem hic modi molestiae ea. Deserunt id vitae ex qui ex facilis. Officia non ullam voluptatem voluptatem sed ratione exercitationem.
Socials
linkedin:
- url : https://linkedin.com/in/jazmin_dev
- username : jazmin_dev
- bio : Excepturi aut deleniti pariatur non earum odit.
- followers : 5670
- following : 534
instagram:
- url : https://instagram.com/jazmin4645
- username : jazmin4645
- bio : Delectus repellat enim et quaerat. Animi inventore debitis rerum. Et rem totam et labore facere.
- followers : 5751
- following : 1930
tiktok:
- url : https://tiktok.com/@jazmin_xx
- username : jazmin_xx
- bio : Aspernatur qui enim nemo iusto temporibus.
- followers : 6850
- following : 14
twitter:
- url : https://twitter.com/jazmin_dev
- username : jazmin_dev
- bio : Dolor dignissimos vitae et dolores soluta. Velit sint in repudiandae saepe omnis itaque. Hic officia vitae nesciunt unde.
- followers : 5108
- following : 1884
facebook:
- url : https://facebook.com/jjacobson
- username : jjacobson
- bio : Architecto alias aliquam sapiente minima assumenda et autem voluptatem.
- followers : 6196
- following : 2303