What does this symbol represent? How does this term impact data analysis and reporting?
The symbol, likely referring to "average," is a common statistical term. It signifies the central tendency of a dataset, calculated by summing all values and dividing by the count. For instance, the average of 2, 4, and 6 is 4. This calculation is fundamental in various fields, from finance to scientific research, enabling data summarization and comparison.
The average's importance lies in its ability to condense complex data into a single, representative value. This allows for easier interpretation and quick comparisons between different sets of data. It is crucial for understanding trends, patterns, and potential outliers within a dataset. Historically, the concept of average has been central to arithmetic and statistics, used in diverse contexts since ancient civilizations.
The understanding of this fundamental statistical concept is essential to proceeding with the main article topics, which likely explore applications of average values in a particular context.
Average
Understanding the concept of average is crucial for data analysis, interpretation, and informed decision-making. Its significance stems from its ability to summarize and compare large datasets.
- Central tendency
- Data summarization
- Trend identification
- Outlier detection
- Comparison analysis
- Statistical inference
- Descriptive statistics
- Probability estimation
These aspects collectively underscore the importance of average in data analysis. Central tendency provides a representative value, while summarization simplifies complex datasets. Identifying trends allows for predicting future outcomes. Outlier detection pinpoints unusual data points. Comparison analysis facilitates evaluating differences across groups. Statistical inference builds models to predict future events. Descriptive statistics describe data distributions. And probability estimation quantifies the likelihood of specific outcomes. For example, the average income of a population reveals economic trends. Likewise, the average temperature over a period indicates climate patterns.
1. Central Tendency
Central tendency, a fundamental concept in statistics, describes the typical or central value within a dataset. The term "average" often serves as a synonym or shorthand for this central value. Central tendency measures the tendency of data values to cluster around a central point. The "average," or mean, is a primary measure of central tendency. Its calculation involves summing all data points and dividing by the total count. A consistent and reliable measurement of the center of a data set is crucial for descriptive statistics and understanding data distributions. Examples range from calculating the average height of individuals within a population to determining the mean temperature over a year, demonstrating its applicability across diverse fields.
The importance of understanding central tendency stems from its ability to simplify and interpret large datasets. A single average value encapsulates crucial information about a data distribution, allowing for quicker comparisons and analyses. This is particularly valuable in areas like economics, where average income figures highlight societal trends and economic disparities. Identifying the average customer satisfaction rating, for instance, enables businesses to gauge their service quality and make crucial improvements. In scientific studies, the average result of an experiment provides a statistically sound estimate of the phenomenon under investigation. This simplification of complex data is paramount for effective communication and actionable insights. Furthermore, comparisons between central tendencies (averages) across different groups or time periods enable us to identify trends and patterns, leading to a deeper understanding of the data's overall narrative. Understanding the variability around this central value, measured by concepts like standard deviation, further enhances the depth of analysis.
Central tendency, epitomized by the "average," is a cornerstone of data analysis. Its proper application fosters a comprehensive understanding of data distributions. By recognizing the central tendency and associated statistics, informed decisions can be made, trends can be identified, and significant patterns can be understood. The practical significance of this understanding lies in its ability to derive meaningful insights from diverse data sets across multiple disciplines, enabling informed decision-making.
2. Data Summarization
Data summarization is a crucial process in data analysis, particularly when dealing with large datasets. It condenses a substantial amount of information into a more manageable form, often using summary statistics like the average. This process simplifies data interpretation and facilitates the identification of key patterns and trends. The average, as a core measure of central tendency, plays a pivotal role in this summarization.
- Simplification of Complex Data
Summarization simplifies complex datasets by reducing the volume of data while retaining key information. Instead of examining individual data points, the average offers a representative value of the data's central tendency. This simplification enables analysts to quickly grasp the general characteristics of the data, such as the typical value or the overall magnitude. For instance, in economic reports, the average income provides a snapshot of the economic health of a region, avoiding the need to examine the income of every individual.
- Facilitating Comparisons
Summary statistics like the average allow for effortless comparisons across different datasets or time periods. Examining average values provides a clear benchmark for evaluating the relative performance or characteristics of distinct groups. In educational contexts, the average test scores across different classes provide a standard for evaluating the effectiveness of teaching methodologies or the academic preparedness of student groups.
- Identifying Patterns and Trends
By condensing data into summary statistics, analysts can more readily identify patterns and trends. Tracking the average sales figures over time, for example, allows businesses to discern seasonal fluctuations or overall growth trends. Similarly, in scientific research, the average response of subjects in an experiment can reveal crucial insights into a phenomenon.
- Improving Data Accessibility
Summarization enhances data accessibility by presenting insights in a concise format. This facilitates easier understanding and interpretation for a broader audience, including stakeholders, decision-makers, and even the general public. Public health reports, for example, often present mortality rates as averages to convey crucial public health information clearly and accessibly.
In conclusion, data summarization, utilizing the average as a primary statistic, is essential for effectively managing and analyzing large datasets. The various facets described simplification, comparison, pattern identification, and improved accessibility demonstrate the critical role summarization plays in extracting meaningful information and driving informed decisions across diverse fields.
3. Trend Identification
Trend identification relies heavily on analyzing average values across time or categories. The average, as a measure of central tendency, provides a critical baseline for detecting shifts or patterns in data. Changes in the average over time, relative to established baselines or previous averages, often signal a trend. For example, observing a consistent upward trend in average sales figures suggests growth or increasing market demand. Conversely, a declining average indicates potential decline or market saturation. This connection is fundamental in many fields.
Identifying trends necessitates analyzing how average values shift over time. Consider stock market analysis: a rising average stock price for a particular company across several quarters may indicate investor confidence and growth prospects. In healthcare, a consistently declining average patient wait time indicates improved operational efficiency. In education, a steady increase in average test scores for a particular student group across multiple assessments might suggest the effectiveness of an educational intervention. These instances demonstrate how trend identification, directly reliant on average values, informs various sectors. Variations from expected averages, either increases or decreases, are particularly significant and require further scrutiny.
Accurate trend identification using average values is essential for informed decision-making. Identifying rising or falling trends allows for proactive strategies. A sustained increase in average online orders might necessitate adjustments to production capabilities. A notable decline in average customer satisfaction scores could prompt investigations into service quality issues. Such insights, rooted in understanding average behavior, enable businesses to adapt, optimize processes, and mitigate risks. A failure to recognize trends, stemming from a lack of attention to average values, can lead to missed opportunities, wasted resources, or potentially detrimental consequences.
4. Outlier Detection
Outlier detection is intrinsically linked to the concept of average. Identifying outliersdata points significantly differing from the typical patternis crucial in analyzing data accurately. The average, representing the central tendency, plays a pivotal role in this process, as outliers often deviate substantially from this central value. This deviation warrants further investigation, as it might signal errors, unusual circumstances, or important insights not captured by the typical data pattern.
- Identifying Data Errors
Outliers can often signify errors in data collection, entry, or measurement. A remarkably high or low value, compared to the established average, might indicate an incorrect measurement, faulty equipment, or human error. For instance, an unusually high temperature reading in a series of weather measurements may indicate malfunctioning equipment or a localized anomaly, not representative of the overall trend. Recognizing these errors allows for their correction, ensuring more accurate and reliable analyses.
- Highlighting Unusual Events
Outliers may also indicate unusual or unexpected occurrences. A sharp increase or decrease in an average value, like sales figures, can often be associated with specific events, promotions, or external factors not reflected in typical patterns. A sudden spike in emergency room visits, compared to the average, could signal an outbreak of a particular illness or a critical incident necessitating immediate attention. These anomalous events warrant further investigation to understand their cause and potential impact.
- Uncovering Important Insights
While outliers often signal errors or anomalies, they can also unveil important insights not otherwise apparent. A seemingly significant outlier in a series of customer satisfaction scores, deviating substantially from the average, could indicate a specific area needing improvement or a segment of customers requiring unique attention. Analyzing these outliers might reveal a previously unrecognized factor influencing customer satisfaction, ultimately leading to targeted interventions and improvements.
- Impact on Average Calculations
Outliers can significantly impact the calculation of the average. A single extreme value can substantially shift the average, potentially misrepresenting the typical data pattern. Recognizing and handling outliers is crucial for maintaining the accuracy and relevance of the average as a descriptive statistic. Techniques such as using median or other robust measures of central tendency can be employed when outliers are likely to skew the average. Understanding this impact is crucial for choosing appropriate methods for data analysis and interpretation.
In conclusion, outlier detection and the concept of average are deeply interconnected. Identifying outliers, based on their deviation from the average, aids in correcting errors, understanding unusual circumstances, uncovering insights, and improving the accuracy of data interpretation. Proper handling of outliers is vital for producing meaningful insights and reliable conclusions from analyzed data, especially when evaluating patterns and trends.
5. Comparison Analysis
Comparison analysis, a fundamental aspect of data interpretation, relies heavily on the concept of average. The average, or mean, provides a standardized metric for comparing different data sets or subgroups. Comparison analysis critically assesses similarities and differences by examining how values deviate from, or converge towards, the average. Understanding this connection is crucial for drawing meaningful conclusions from data. For example, comparing average income levels across different demographics reveals socioeconomic disparities and facilitates informed policy decisions.
Effective comparison analysis requires a clear understanding of the average across various groups or time periods. Differences in averages often highlight significant patterns or trends. In business, comparing average sales figures across different marketing campaigns provides insights into campaign effectiveness and allows for optimization. Similarly, comparing average patient recovery times across different treatment protocols allows medical professionals to identify more efficient and effective strategies. In educational settings, comparing average test scores among students from varying socioeconomic backgrounds reveals educational disparities and aids in targeted interventions. Statistical significance testing further reinforces the reliability of these comparative analyses, by accounting for the inherent variability within the datasets.
By analyzing how different groups or situations relate to an average, comparison analysis uncovers meaningful patterns and insights. The average serves as a crucial reference point, allowing for a comprehensive understanding of differences in performance, characteristics, or outcomes. Such analysis facilitates informed decision-making across numerous fields, from business strategy to public policy. Challenges might arise in situations with extremely skewed data distributions or when comparing incomparable data sets. Careful consideration of the data's characteristics, including potential outliers, is essential to ensure the accuracy and validity of the comparisons made using averages. However, leveraging the average in comparison analysis remains a vital tool for gaining meaningful and actionable insights from diverse data.
6. Statistical Inference
Statistical inference draws conclusions about a population based on data collected from a sample. The sample average, a crucial element in statistical inference, serves as an estimator for the population average. The validity and reliability of the inferences hinge on the accuracy and representativeness of this sample average. Using the sample average to estimate the population average relies on principles of probability and sampling distributions. In essence, statistical inference uses the sample average as a tool to make generalizations about the entire population.
The importance of statistical inference in understanding averages extends across various fields. For instance, in medical research, determining the average effectiveness of a new drug requires inferential statistics. By analyzing data from a sample of patients, researchers can estimate the true average effect of the drug on the entire population. Similarly, in marketing, analyzing sales figures from a sample of customers allows businesses to infer the true average purchase amount for their entire customer base. In each case, statistical inference provides a means to extrapolate from limited data to a larger, more generalizable context. Statistical measures such as confidence intervals quantify the uncertainty associated with these inferences, providing a range of plausible values for the population average. The larger the sample size and the less variability within the data, the more precise the inference.
In summary, statistical inference is inextricably linked to the concept of the average. The sample average serves as a cornerstone for estimating the population average. The rigor of the inferential process ensures that generalizations about population averages are not only accurate but also account for the inherent variability in data. Through proper application of statistical methods, researchers and analysts can confidently derive insights from their data, making informed decisions and predictions. Understanding the principles underlying statistical inference strengthens the reliability and validity of the conclusions drawn about average values.
7. Descriptive Statistics
Descriptive statistics are fundamental tools for summarizing and presenting data. The average ("$avge") is a critical component of descriptive statistics, providing a measure of central tendency. Understanding how descriptive statistics utilize the average helps in effectively communicating and interpreting data insights.
- Central Tendency Measures
Descriptive statistics employ various measures of central tendency, with the average ("$avge") as a prominent example. The average (or mean) represents the typical value within a dataset. In business, the average sales figures across various product lines offer insights into general market trends. The average customer satisfaction rating aids in assessing a company's performance and identifying areas for improvement. This metric, and other central tendency measures like median and mode, summarize data effectively, highlighting the typical behavior within a dataset.
- Variability Measures
Alongside central tendency, descriptive statistics also evaluate data variability. Measures like standard deviation, range, and interquartile range provide insights into how spread out data points are from the average ("$avge"). A low standard deviation indicates data points are clustered closely around the average, suggesting high consistency. Conversely, a high standard deviation signifies greater data dispersion. For example, analyzing the standard deviation of student scores on a test provides information about the homogeneity or heterogeneity of the student group. A high standard deviation might indicate a need for varied instructional strategies, whereas a low standard deviation suggests the current approach is largely effective.
- Frequency Distributions
Descriptive statistics can display data using frequency distributions. These distributions present the frequency of occurrence for different values or ranges of values. The average ("$avge") often forms a crucial reference point in these distributions. For example, in a study of ages of people attending a concert, a frequency distribution graph would present how many people fall into age ranges. The average age would be a point of reference, allowing visual identification of the age range with the highest concentration of attendees.
- Data Visualization
Descriptive statistics extensively utilize data visualization techniques like histograms, box plots, and scatter plots. Visual representations help to quickly comprehend data patterns. Visualizing the average ("$avge") within these charts adds context. By displaying the average along with the distribution, users can immediately assess the central tendency relative to the range of data. Analyzing a scatter plot with the average revenue plotted against sales volume allows for assessing correlations and patterns.
In conclusion, descriptive statistics, using the average ("$avge") as a cornerstone, provide a structured and comprehensive way to understand and communicate data insights. Understanding central tendency and variability through various measures and visualizations allows users to gain accurate and valuable insights from the data. This, in turn, facilitates informed decision-making and improved understanding of the underlying trends or patterns within the data.
8. Probability Estimation
Probability estimation, a core concept in statistics, often relies on understanding average values. The average, representing the central tendency of a dataset, forms a foundation for estimating the likelihood of specific events or outcomes. In essence, the average acts as a benchmark for judging the probability of a particular data point occurring within a dataset. A data point significantly higher or lower than the average might suggest a lower probability of occurrence. Consider the average height of individuals in a population; a probability estimate can be derived concerning the likelihood of an individual being above or below that average height.
The practical significance of this connection is substantial. For instance, in financial modeling, predicting future stock prices often incorporates probability estimation based on historical average price movements. Understanding the average return on investment across various market sectors, along with associated probabilities, informs investment strategies. In medical research, the average recovery time for a particular treatment significantly informs the probability estimation concerning successful treatment outcomes. Similarly, in manufacturing, the average defect rate in a production run aids in assessing the probability of a defective product emerging. In each case, the average forms a crucial component in assessing the probability of a certain outcome.
In conclusion, probability estimation and average values are intrinsically linked. The average acts as a key reference point for estimating probabilities within a dataset. Understanding this connection enables informed decision-making in various contexts. However, limitations exist in relying solely on averages to estimate probabilities, especially when dealing with complex datasets or situations with significant deviations from the typical pattern. Acknowledging these limitations enhances the validity and accuracy of probability estimations derived from average values.
Frequently Asked Questions about Average
This section addresses common questions regarding the concept of average, a fundamental statistical measure. Answers are provided in a clear and concise manner, focusing on accuracy and clarity.
Question 1: What is the meaning of "average"?
Average, in a statistical context, represents the central tendency of a dataset. It is calculated by summing all values in a set and then dividing by the total number of values. This single value summarizes the data's central point, providing a quick overview of the data's distribution.
Question 2: How is the average calculated?
The calculation involves summing all the data points and then dividing the sum by the total number of data points. For example, the average of 2, 4, 6, and 8 is (2 + 4 + 6 + 8) / 4 = 5.
Question 3: What are the limitations of using the average?
The average can be sensitive to extreme values, also known as outliers. A single outlier can significantly alter the calculated average, potentially misrepresenting the data's overall characteristics. The median, a different measure of central tendency, can be more robust in the presence of outliers. Furthermore, averages do not capture the entire distribution of the data, and further statistical analysis might be needed to get a full picture.
Question 4: When is the average a useful measure?
The average is valuable when data points tend to cluster around a central value, providing a representative summary of the data's central tendency. It's beneficial for comparisons across different datasets or groups, enabling swift assessments and identifying general trends. The average is a useful starting point, but often it is necessary to supplement this with other statistical measures to fully understand the dataset.
Question 5: How can I interpret the average in context?
Interpreting the average requires considering the context of the data. For example, the average income of a population can reveal economic trends, while the average test score in a class can indicate the effectiveness of teaching methodologies. Analyzing the context of the data is crucial, ensuring the appropriate interpretation of the calculated average.
In summary, the average is a valuable statistical tool for understanding central tendencies within datasets, but it should be considered alongside other descriptive statistics to gain a comprehensive understanding of the data. Recognizing limitations, such as the effect of outliers, and applying the average within a meaningful context are essential for drawing accurate conclusions.
This concludes the FAQ section. The next section will delve deeper into specific applications of average calculations.
Conclusion
This exploration of "average" ("$avge") reveals its fundamental role in data analysis and interpretation. The average, as a measure of central tendency, simplifies complex data by condensing it into a single representative value. This simplification facilitates comparisons across different datasets, identifies trends over time, and aids in outlier detection. Crucially, the average's application is not limited to a single field; its utility spans diverse domains, from business analysis and medical research to public policy and scientific inquiry. Key aspects elucidated include its role in summarization, trend identification, outlier detection, comparison analysis, statistical inference, and descriptive statistics, each highlighting the average's importance in extracting meaningful insights.
The average provides a critical baseline for understanding data distributions. While acknowledging potential limitations, such as susceptibility to outliers, this study underscores the importance of a thorough understanding of average calculation and interpretation. Accurate and informed decision-making relies on recognizing the nuances and implications of employing this fundamental statistical concept. Consequently, continued exploration and refinement in applying average calculations will contribute to deeper insights and more effective analyses in various fields.

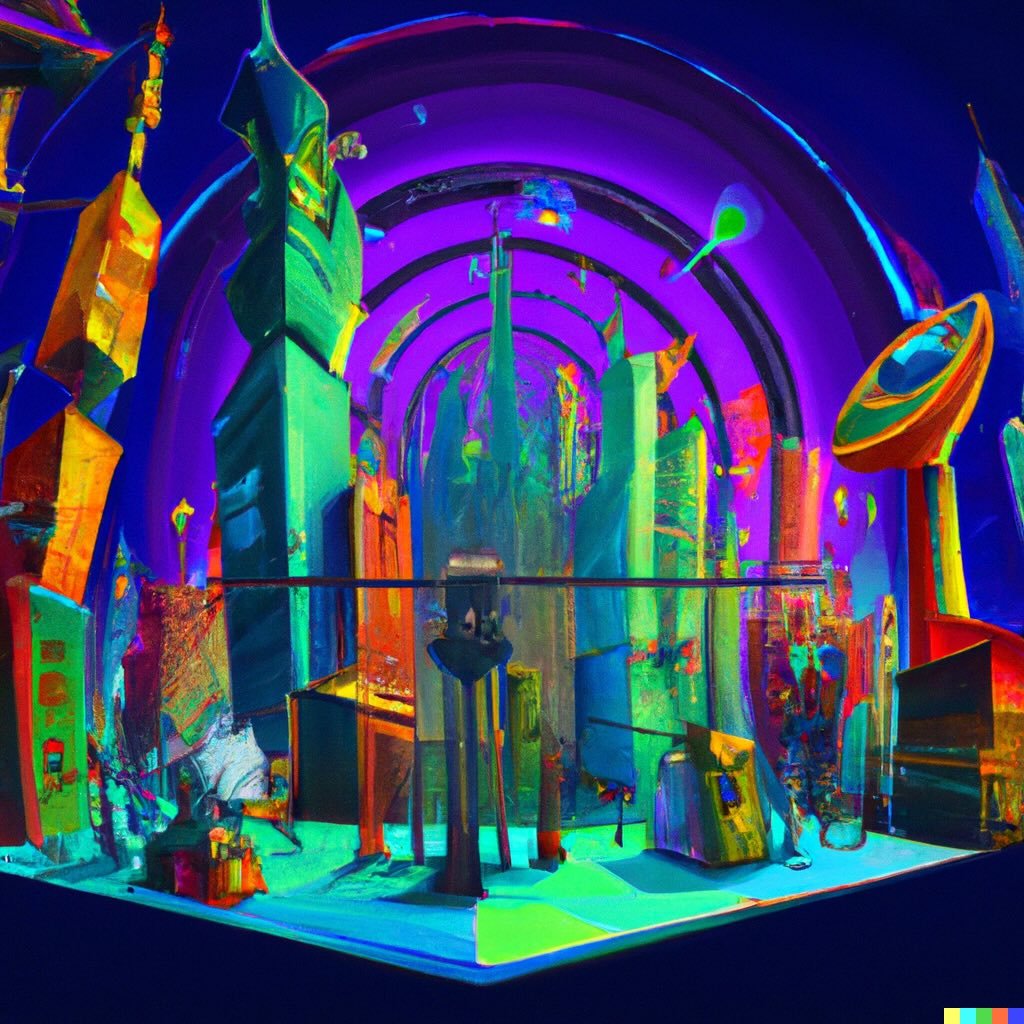
Detail Author:
- Name : Alexis Grady
- Username : ctorphy
- Email : julien50@gmail.com
- Birthdate : 1993-07-28
- Address : 26253 Will Vista Suite 454 New Misaelton, LA 59988-7264
- Phone : (219) 617-8260
- Company : Marvin-Oberbrunner
- Job : Timing Device Assemblers
- Bio : Rerum maiores quo distinctio quaerat labore distinctio possimus. Rerum delectus iusto sunt enim voluptas. Aut temporibus tempore quod optio eum ex voluptates.
Socials
linkedin:
- url : https://linkedin.com/in/sawayn2000
- username : sawayn2000
- bio : Laboriosam quo veniam facere.
- followers : 228
- following : 611
tiktok:
- url : https://tiktok.com/@sawayn2001
- username : sawayn2001
- bio : Autem sit tempora a facilis earum explicabo assumenda.
- followers : 4968
- following : 757