What is this crucial concept? How does it impact our understanding of a specific subject area?
This method, central to statistical analysis, quantifies the association between variables. It assesses the degree to which paired observations tend to move together or diverge. For example, analyzing the relationship between crop yields and rainfall might employ this approach to determine if they are positively correlated. The output, often expressed as a numerical value, indicates the strength and direction of the relationship.
This approach is valuable because it provides a concise summary of the degree of association. This simplification aids interpretation and facilitates the comparison of various datasets or relationships. Its use in research across multiple disciplines, including economics, sociology, and environmental science, underscores its importance. The historical development of statistical methods and its evolution show its continued relevance to contemporary issues.
Moving forward, this article will delve into the practical application of this method in various fields and its relevance in contemporary research.
Anscor
Understanding anscor involves recognizing its multifaceted nature. The core elements of anscor provide a structured approach for analyzing relationships between variables in various disciplines.
- Correlation
- Statistical
- Relationship
- Quantification
- Interpretation
- Comparison
- Dataset
- Variables
The key aspects of anscorcorrelation, statistical relationship, quantification, and interpretationtogether form a powerful analytical tool. This tool enables researchers to understand how variables interact. For example, comparing crop yields with different rainfall amounts through an anscor analysis allows quantification and interpretation of the correlation between these variables. Likewise, in economic studies, the statistical relationship between investment and economic growth can be assessed using similar methods. Dataset comparisons and analyses help in making decisions and understanding diverse patterns.
- Spacious Acreage Brewery Craft Brews Stunning Views
- Verizon Service In Kilmarnock Va Reliable Phone Internet
1. Correlation
Correlation, a fundamental concept in statistical analysis, forms a crucial component of the methodology often referred to as "anscor." Understanding correlation is essential for interpreting and evaluating the results generated by this approach.
- Definition and Measurement
Correlation quantifies the degree and direction of a relationship between two variables. A positive correlation indicates that as one variable increases, the other tends to increase as well. Conversely, a negative correlation suggests that as one variable increases, the other tends to decrease. Various statistical measures, such as Pearson's correlation coefficient, quantify this relationship, providing a numerical value ranging from -1 to +1 to describe the strength and direction of the association.
- Types of Correlation
Different types of correlation exist, reflecting varying degrees and kinds of association. Linear correlations describe relationships that follow a straight line pattern. Non-linear correlations depict more complex associations that deviate from a straight line. Understanding the specific type of correlation helps contextualize the findings when applied to "anscor" methodology and subsequent interpretations.
- Applications in "anscor" Analysis
The application of correlation within "anscor" methodologies involves examining how paired data points relate to each other. For instance, assessing the correlation between rainfall and crop yield in agricultural research exemplifies a use case. In financial analysis, correlation can reveal connections between stock prices and market trends. The insights derived inform decisions and interpretations in "anscor" analysis by quantifying these associations.
- Interpreting Correlation Coefficients
The value of the correlation coefficient provides specific insights into the nature of the relationship. A coefficient of zero indicates no linear relationship. Coefficients approaching 1 suggest a strong linear relationship, while values closer to zero indicate a weak relationship. This interpretation becomes critical within the "anscor" framework, where decisions are influenced by the strength and direction of identified relationships between variables.
In summary, correlation is a foundational element of "anscor." The methods used to measure correlation, the different types of correlation, and the implications for analysis all play crucial roles in the context of a broader "anscor" methodology. The detailed quantification and understanding of correlation are essential to proper interpretation within the overall framework.
2. Statistical
Statistical methods underpin the analysis often described as "anscor." The core principles of statistical reasoning are indispensable for extracting meaningful insights from data and understanding relationships between variables. Statistical concepts such as correlation, regression, and hypothesis testing are integral to the methodology, enabling rigorous assessment of observed patterns. This approach ensures the findings derived from "anscor" are grounded in quantifiable evidence and not arbitrary subjective opinions.
- Descriptive Statistics
Descriptive statistics summarize and present data characteristics. Measures like mean, median, and standard deviation provide a concise picture of the central tendency and variability within datasets. In "anscor," descriptive statistics lay the groundwork for subsequent analyses by highlighting key features of the data. For instance, in agricultural research, descriptive statistics might summarize crop yields across various regions, enabling comparisons and guiding further investigation.
- Inferential Statistics
Inferential statistics extend beyond description, using sample data to draw inferences about a larger population. Techniques such as hypothesis testing evaluate whether observed relationships are statistically significant, rather than due to random chance. This is crucial in "anscor" analysis, allowing conclusions to be generalized beyond the specific dataset examined. Analyzing the correlation between two variables using these inferential techniques in "anscor" establishes whether the relationship is likely to hold true in a broader context.
- Probability and Distributions
Probability principles and distributions provide the theoretical framework for understanding the variability inherent in data. Statistical models, which draw on probability distributions, estimate the likelihood of various outcomes and the confidence intervals associated with estimations. The quantification of uncertainty through probability and distributions is vital in "anscor" analysis for drawing reliable conclusions from data.
- Correlation and Regression Analysis
Correlation and regression methods, frequently employed in "anscor," quantify and model the relationships between variables. Correlation assesses the strength and direction of the association, while regression models the relationship in a predictive manner. These tools in "anscor" are vital for identifying causal or associated trends and establishing the strength of observed relationships between variables, such as the correlation between rainfall and crop yield.
In essence, the statistical framework provides a rigorous, objective, and measurable structure for "anscor." The application of statistical tools empowers the analysis to determine not only the presence of a relationship but also the statistical significance and reliability of that relationship.
3. Relationship
The concept of "relationship" is central to "anscor." This method fundamentally examines the nature and strength of associations between variables. A robust "relationship" analysis is critical for understanding how changes in one variable might influence another. This understanding is crucial across diverse fields, from agricultural research analyzing crop yields and rainfall to financial modeling assessing stock market trends. For instance, a strong "relationship" between rainfall and crop yields might indicate the importance of adequate water for agricultural output. Likewise, a strong negative "relationship" between interest rates and consumer spending signifies a potential economic impact.
The strength and direction of these "relationships" are quantified. This quantification is critical for making informed decisions. In agricultural practices, the analysis could inform irrigation strategies, while in finance, it might guide investment portfolios. These quantifiable insights inform strategies based on a clear understanding of interdependencies. The identification and quantification of "relationships" through "anscor" methodologies allow for predictive modeling. Accurate predictions are essential across diverse sectors, from forecasting crop yields for effective resource allocation to evaluating investment strategies and risk management in finance.
In essence, "relationship" analysis, as a core component of "anscor," provides a structured and measurable approach to understanding the connections between variables. The insights derived inform better decision-making and allow for more effective strategies in various fields. The importance of "relationship" within the "anscor" framework lies in its ability to provide not only a description but also a predictive capacity for informed decisions and effective strategies.
4. Quantification
Quantification is integral to "anscor" analysis. The process of assigning numerical values to observed phenomena allows for precise measurement and comparison of relationships between variables. This numerical representation facilitates the identification of patterns and trends, providing a robust foundation for drawing inferences and ultimately making informed decisions. For instance, in agricultural research, the quantification of rainfall levels and subsequent crop yields allows for a precise assessment of the impact of rainfall on agricultural output. Similarly, in financial markets, the quantification of various economic indicators enables the assessment of correlations and potential risks.
The crucial role of quantification in "anscor" lies in its ability to objectify analysis. Instead of relying on subjective assessments, numerical data enables an objective comparison and evaluation of relationships. This objectivity is particularly valuable in fields like science and finance, where decisions must be grounded in verifiable data. Precise numerical measurements ensure consistency and reproducibility, allowing different researchers or analysts to reach similar conclusions when analyzing comparable data. Furthermore, quantification enables statistical modeling and prediction. The numerical representation allows for the development of mathematical models that can accurately reflect the relationship between variables and predict future outcomes. This capability is particularly valuable in forecasting crop yields or assessing financial risks.
In essence, quantification provides the language of "anscor." It transforms qualitative observations into measurable data, enabling a structured and rigorous approach to identifying, analyzing, and interpreting relationships between variables. This allows for a consistent and replicable methodology across different contexts. By assigning numerical values, "anscor" transcends subjective interpretations, establishing a firmer basis for reliable conclusions and informed decisions. The significance of quantification in "anscor" ultimately lies in its ability to transform raw data into actionable knowledge, facilitating effective strategy and decision-making across various sectors.
5. Interpretation
Interpretation is not merely a step following "anscor" analysis; it is an inextricable component integral to its very purpose. The numerical data produced by "anscor" methods, such as correlation coefficients or regression models, require interpretation to be meaningful. These quantitative results, without context or explanation, remain isolated data points. Interpretation transforms these figures into actionable knowledge, allowing the analyst to understand the implications of the discovered relationships. Consider, for example, a "anscor" analysis revealing a strong positive correlation between fertilizer application and crop yields. This numerical correlation, without interpretation, provides limited insight. Interpreting this finding as indicating that increased fertilizer application correlates with higher yields allows for agricultural strategies to be developed to improve production.
Practical applications of interpretation in "anscor" extend across many fields. In financial analysis, interpreting a negative correlation between stock prices and interest rates suggests a potential inverse relationship. Such interpretation allows investors to develop investment strategies that consider these potential opposing forces. In public health research, an interpreted "anscor" analysis might highlight a correlation between air quality and respiratory illnesses, potentially informing public health policies to mitigate pollution levels. The effectiveness of any "anscor" analysis hinges directly on the quality of the interpretation, translating complex relationships into actionable insights.
In summary, interpretation acts as the bridge between raw data and practical application in "anscor." Without clear, thoughtful interpretation, the numerical results yielded by "anscor" analysis remain essentially useless. A sophisticated understanding of the implications and potential consequences of the data is critical. The ability to draw reasoned conclusions, make accurate predictions, and formulate practical strategies hinges entirely on the quality of interpretation in the context of the "anscor" methodology. Challenges in interpretation often arise from nuanced relationships or complex datasets, underscoring the necessity of rigorous methodologies and consideration of multiple factors during the analysis. Successful "anscor" analysis demands a sharp focus on interpretation and its critical role in translating statistical relationships into meaningful insights for decision-making.
6. Comparison
Comparison is a critical component of "anscor" analysis. The ability to compare datasets, variables, and their relationships is fundamental to extracting meaningful insights and drawing valid conclusions. By comparing different scenarios, researchers can identify patterns, quantify differences, and determine the significance of observed relationships. This process underpins informed decision-making across numerous disciplines.
- Dataset Comparison
Comparing datasets allows for the identification of commonalities and distinctions in different populations or circumstances. For example, analyzing crop yields across different regions under various environmental conditions allows researchers to ascertain how different factors (like soil type or rainfall) affect outcomes. This comparative approach in "anscor" analysis helps understand which factors are most strongly linked to the desired outcome.
- Variable Comparison
Comparing variables allows for a focused examination of relationships. A comparison of the correlation between rainfall and crop yield with the correlation between temperature and yield helps isolate the impact of specific factors. This aspect of "anscor" facilitates identifying primary drivers and disentangling complex relationships, leading to more nuanced conclusions.
- Relationship Strength Comparison
Comparison of the strength and direction of relationships across various datasets or scenarios provides crucial context. Comparing the correlation between different variables affecting stock prices in different market conditions, for instance, allows researchers to determine how these relationships behave in response to distinct economic factors. This comparative perspective is essential in identifying consistent patterns and pinpointing potential vulnerabilities or opportunities.
- Methodological Comparison
Comparative analysis extends to methodological comparisons. Analyzing how different "anscor" methods address similar research questions or different datasets helps in selecting the most appropriate technique for the given context. Assessing the robustness of results across various approaches leads to greater confidence in conclusions and ensures that insights are well-grounded in the principles of data analysis.
Through meticulous comparison, "anscor" analysis unveils critical connections between different variables, datasets, and methodologies. This comparative examination leads to a richer understanding of the relationships under investigation and enables the identification of crucial distinctions between different situations or data samples. Ultimately, a comparative approach strengthens the robustness and reliability of the analysis, allowing for more effective decision-making and a deeper comprehension of complex phenomena.
7. Dataset
A dataset forms the bedrock of "anscor" analysis. The quality, representativeness, and appropriateness of the data directly impact the reliability and validity of any conclusions drawn. "Anscor" methodology relies on data sets for the quantification and interpretation of relationships between variables. Without a robust dataset, the results of "anscor" analysis are fundamentally flawed and potentially misleading. For instance, an analysis of the relationship between advertising expenditure and sales would yield unreliable results if the dataset contained inaccurate or incomplete advertising figures.
The importance of a well-structured dataset extends beyond its accuracy. Representative sampling is critical. A sample that inadequately reflects the broader population will lead to biased results, which may misrepresent the true relationship between variables. For example, a study evaluating the correlation between education level and income, relying on a dataset of only individuals with graduate degrees, would not accurately portray the relationship in the general population. Data sets must be relevant to the research question, avoiding extraneous variables that could mask the true relationships. This includes verifying that the data is free from significant errors and inconsistencies. In agriculture, a dataset of crop yields including data from locations exposed to unusual environmental conditions will skew the correlation of yield versus rainfall and therefore the effectiveness of the study.
Understanding the relationship between datasets and "anscor" is essential for researchers in diverse fields. Ensuring the data's quality, representativeness, and relevance directly affects the validity and applicability of findings. A meticulous approach to data collection, management, and selection is crucial for accurate analysis and well-founded conclusions. Recognizing the limitations inherent in specific datasets or potential biases in the data selection process enhances critical analysis and helps avoid misinterpretations that may arise from incomplete or flawed data. Accurate, comprehensive datasets are indispensable for generating reliable and trustworthy outcomes in "anscor" analyses, irrespective of the specific field of study.
8. Variables
Variables are fundamental components of "anscor" analysis. Their characteristics and interrelationships are central to understanding patterns and drawing conclusions from data. The types and properties of variables significantly influence the methodologies employed and the interpretations derived from "anscor" analysis.
- Data Type and Measurement Scale
Different variables possess distinct data types (numerical, categorical, ordinal). The measurement scale (nominal, ordinal, interval, ratio) significantly impacts the appropriate statistical methods for analysis. For example, analyzing the correlation between income (ratio scale) and education level (ordinal scale) requires different statistical techniques compared to analyzing the association between preferred colors (nominal scale) and satisfaction levels (ordinal scale). Selecting the right method in "anscor" analysis depends on the appropriate understanding of variables' data types and the appropriate numerical scale.
- Independent and Dependent Variables
Identifying independent and dependent variables is crucial in "anscor" analysis. An independent variable influences a dependent variable. For example, in agricultural research, fertilizer application (independent variable) might influence crop yield (dependent variable). Understanding this distinction is essential for interpreting the direction and strength of observed relationships within the "anscor" framework. The type and nature of the independent and dependent variables impact the methodology of correlation and causation assessment within an "anscor" analysis.
- Confounding Variables
Confounding variables can obscure the true relationship between independent and dependent variables. These variables might influence both the independent and dependent variables, leading to misleading conclusions. For example, in studies correlating exercise and health outcomes, socioeconomic status might be a confounding variable. Researchers must control for or account for confounding variables in "anscor" analysis to avoid misinterpretations and draw accurate conclusions.
- Interaction Effects
Some variables might interact to influence the dependent variable. For example, in educational studies, both socioeconomic background and teacher quality might influence student achievement. Understanding potential interaction effects is critical to a comprehensive "anscor" analysis. Researchers need to recognize and account for such interactions to accurately capture the complex interplay between variables in their analysis.
In essence, variables are not passive elements in "anscor." Their types, relationships, and potential interactions significantly shape the analysis and interpretation. A careful consideration of variables, their properties, and potential interdependencies is fundamental to a robust and reliable "anscor" analysis, allowing for accurate assessment and insightful conclusions across diverse fields of study.
Frequently Asked Questions about "Anscor"
This section addresses common inquiries regarding the "anscor" methodology. Clear and concise answers aim to dispel any ambiguities and provide a practical understanding of the core concepts.
Question 1: What is "anscor" and what is its purpose?
"Anscor" represents a set of statistical methods used to quantify and interpret relationships between variables. Its purpose is to identify patterns and correlations within datasets, facilitating deeper understanding of complex phenomena. This method aids in predicting outcomes and informing decisions based on data-driven insights.
Question 2: How does "anscor" differ from other correlation methods?
"Anscor" methodologies employ specific statistical techniques. While similar to other correlation methods, its unique approach allows for more nuanced analyses of complex datasets. Key differences might lie in the specific measures employed and the way data is handled.
Question 3: What types of data are suitable for "anscor" analysis?
"Anscor" analysis is applicable to diverse datasets. However, the selection and quality of data are paramount for reliable results. Variables need to be clearly defined, and the data itself needs to be relevant, consistent, and representative of the phenomenon being studied.
Question 4: What are the limitations of "anscor" analysis?
Like any analytical method, "anscor" possesses limitations. Correlation does not imply causation; "anscor" methods can identify associations but not definitively prove cause-and-effect relationships. The quality and representativeness of the dataset significantly affect the validity of conclusions.
Question 5: How can I ensure the accuracy of "anscor" analysis results?
Rigorous data collection and careful selection are essential. Researchers should thoroughly understand the variables involved, account for potential confounding factors, and utilize appropriate statistical techniques for the data type. A transparent description of methods is vital for evaluating the validity of findings.
In summary, "anscor" provides a valuable framework for data analysis, but its effective application hinges on accurate data, appropriate methods, and a thorough understanding of the limitations. Understanding these aspects will allow informed application and interpretation of findings.
The subsequent section will explore the practical applications of "anscor" in specific fields.
Conclusion
This exploration of "anscor" methodology reveals its multifaceted nature. Central to the process is the quantification and interpretation of relationships between variables. The analysis hinges on the quality and representativeness of the dataset, the careful selection and understanding of variables, and a rigorous application of statistical methods. Key components, including correlation, statistical principles, and the interpretation of relationships, collectively contribute to the strength and reliability of the findings. The analysis aims to provide a structured, quantitative approach for understanding complex phenomena across diverse disciplines, from agriculture to finance.
The methodology's strength lies in its ability to provide objective and measurable insights into complex relationships. However, limitations exist, particularly in establishing causality based solely on correlations. Careful interpretation, addressing potential confounding variables, and a thorough understanding of the limitations are essential for meaningful application. Further research could explore the development of more sophisticated analytical techniques within the framework of "anscor" to address these limitations and enhance its predictive capabilities. This rigorous methodology holds significant potential for informing decision-making and advancing knowledge across various fields of study. Continued development and application of "anscor" will be crucial in navigating the complexities of contemporary issues.
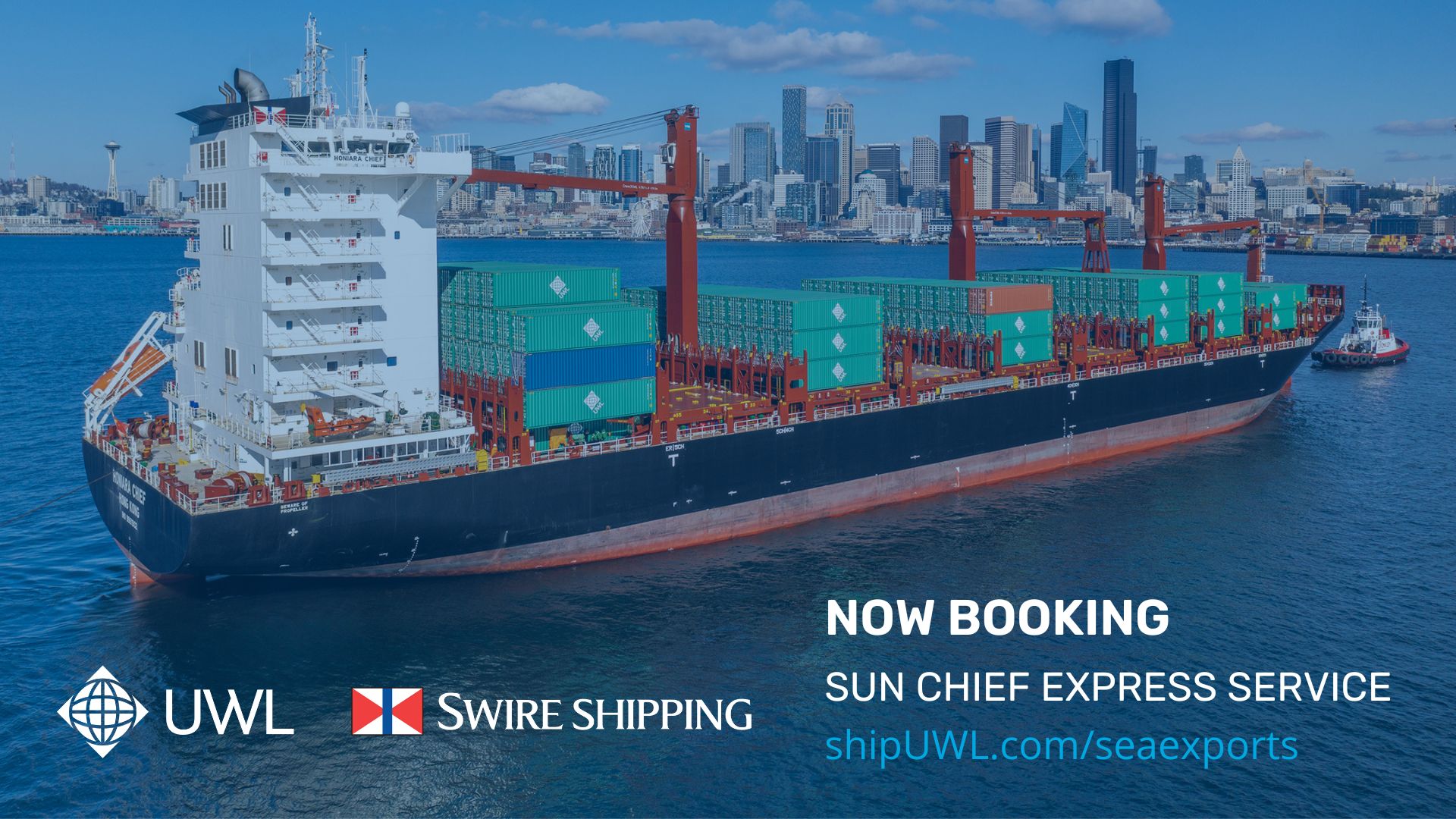
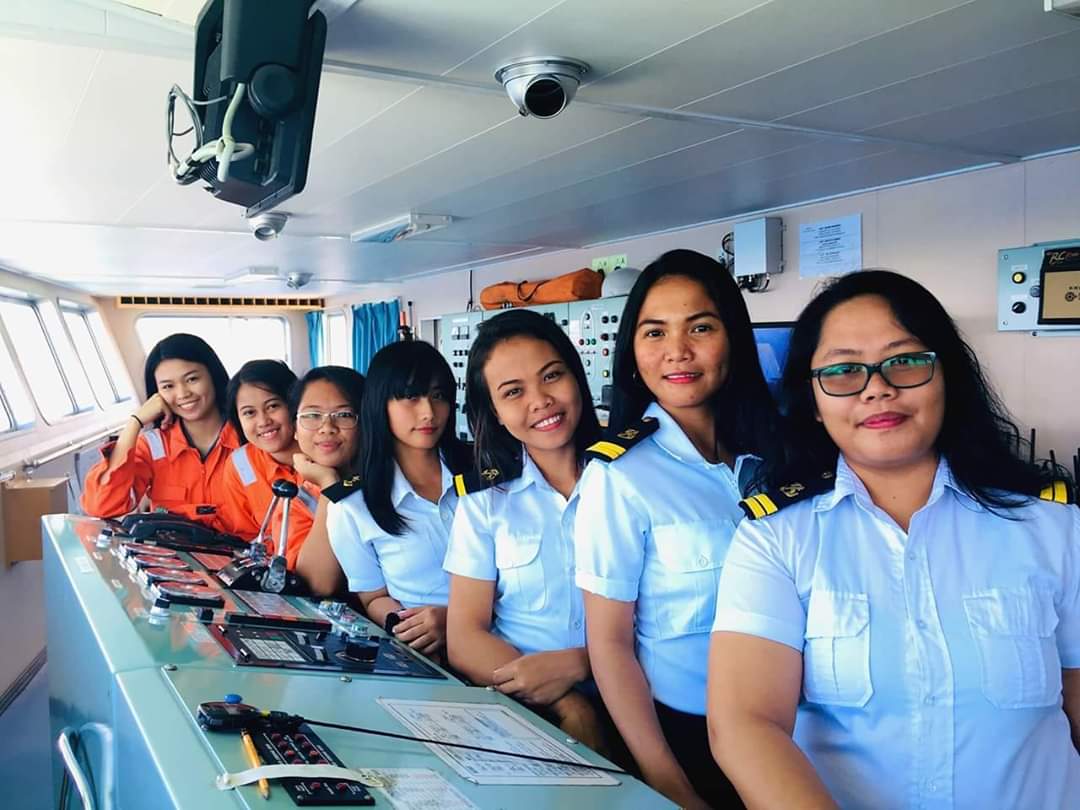

Detail Author:
- Name : Ashlee Effertz
- Username : bernhard.stephany
- Email : karianne.carroll@gmail.com
- Birthdate : 1975-01-10
- Address : 115 Dare Lights Suite 150 Streichhaven, LA 92243
- Phone : (585) 213-0952
- Company : Klein Ltd
- Job : Geoscientists
- Bio : Est odit voluptas eius illum sunt vero eum eos. Ea distinctio quo accusamus sit voluptas amet illo. Earum debitis et ab neque eligendi. Veritatis provident dolorum tempora facilis autem corrupti.
Socials
instagram:
- url : https://instagram.com/luettgenr
- username : luettgenr
- bio : Nesciunt et porro corporis hic. In a ducimus animi eveniet necessitatibus numquam.
- followers : 5710
- following : 2224
facebook:
- url : https://facebook.com/rayluettgen
- username : rayluettgen
- bio : Quibusdam numquam vel dolorum. Quis nam sed nulla fugiat consequatur ea.
- followers : 6577
- following : 742