What are the implications of this particular data stream, and how does it influence various fields? This data stream offers unique insights into...
This data stream represents a continuous flow of information, specifically structured and collected in a manner that enables detailed analysis of a particular domain. For example, it might track real-time user engagement patterns on a social media platform, or capture detailed financial transactions from a specific geographic region. The structure of this data stream is crucial; it needs clear definitions, consistent formats, and appropriate tagging to facilitate meaningful interpretation. It's not simply raw data, but rather a curated flow of information, valuable for extracting trends, understanding behavior, and informing decision-making.
The importance of this type of data stream lies in its ability to provide real-time insights into complex systems. Analysis of this stream can reveal previously unidentified trends, allowing for adaptive responses and optimized strategies across various sectors. This information, when effectively processed, can inform critical decisions in areas like market prediction, resource allocation, and public health monitoring. The potential for improvement in predictive modeling and operational efficiency, made possible through this structured data flow, is significant.
- Former President Trumps Signature Authentic Examples Analysis
- Ibrahim Hegab Top Insights Expert Advice
Moving forward, the analysis of this continuous flow of data will be explored in more depth, focusing on the diverse methodologies used in gathering and processing this stream of information, and ultimately understanding its application in a number of fields. Specific examples of its practical use cases across different industries will be discussed, including its impact on financial markets and its significance in public policymaking.
Habs Stream
Understanding the core components of a data stream, particularly a "habs stream," is crucial for interpreting and applying its insights. This structured flow of information holds significant potential across various domains.
- Data Collection
- Format Consistency
- Real-time Analysis
- Pattern Identification
- Predictive Modeling
- Decision Support
- Scalability
The "habs stream," a continuous data flow, relies on consistent data collection methods for accurate analysis. Format consistency allows for seamless processing and real-time interpretation, enabling the identification of meaningful patterns. This real-time analysis, in turn, facilitates predictive modeling and supports informed decisions. The importance of scalability is also crucial; a system capable of adapting to increasing data volumes is vital for long-term effectiveness. The ability to adapt and use a "habs stream" for decision support in diverse fields, from finance to healthcare, underscores its significant implications.
1. Data Collection
Accurate and efficient data collection forms the bedrock of a "habs stream." The quality and reliability of the stream's output directly correlate to the rigor of the collection process. This involves meticulously defining the data points to be captured, establishing standardized methods for acquisition, and implementing safeguards against errors and biases. Without a robust collection process, the stream's value is compromised, hindering effective analysis and potentially leading to inaccurate conclusions. For instance, in financial markets, a "habs stream" tracking transaction data relies entirely on precise, real-time recording of trades. Imperfect or delayed data collection could lead to flawed market predictions and potentially significant financial losses.
The importance of data collection extends beyond simply gathering figures. The structure and context within which data is collected are equally significant. For example, in a "habs stream" designed to monitor social media sentiment, the collection process must specify the sources (platforms, keywords, etc.) to ensure the data accurately reflects the intended target. Similarly, in healthcare, a "habs stream" for patient data necessitates meticulous record-keeping, ensuring consistency across different departments to avoid conflicts in interpretation. Effective data collection requires clear definitions, comprehensive documentation, and meticulous adherence to pre-defined parameters to accurately reflect the nuanced reality the stream is designed to capture. The more precisely and thoroughly the data is collected, the more reliable and actionable the "habs stream" will be.
In conclusion, data collection is not merely a precursor to a "habs stream"; it is its very essence. A comprehensive understanding of the collection methodology, including its limitations and potential sources of bias, is critical to utilizing the data stream effectively. Addressing the challenges associated with data quality and consistency is essential to extracting meaningful insights from the "habs stream" and achieving its intended purpose. This necessitates a proactive, ongoing process of review and refinement to ensure the ongoing accuracy, consistency, and usefulness of the "habs stream."
2. Format Consistency
Maintaining consistent data formats is fundamental to the efficacy of a "habs stream." A standardized format enables reliable interpretation, analysis, and integration of data points within the stream. Inconsistencies introduce complexities, hindering the ability to extract meaningful insights and potentially leading to erroneous conclusions. The importance of format consistency cannot be overstated in ensuring the "habs stream" functions as an effective tool for data-driven decision-making.
- Data Structure Definition
Precise definitions and specifications for each data element within the "habs stream" are paramount. This includes meticulously defining the types of data (e.g., numeric, string, date), their lengths, allowed values, and units of measurement. A well-defined structure ensures that data points are uniformly categorized, enabling the use of standardized algorithms and analysis tools, minimizing the likelihood of errors. For instance, a "habs stream" tracking financial transactions must precisely define the structure for fields like transaction date, amount, and account numbers to prevent misinterpretations of financial activities.
- Field Naming Conventions
Establishing consistent naming conventions across all data points within the "habs stream" is vital. Clear and standardized names for fields eliminate ambiguity and ensure accurate data interpretation. This convention must be consistently applied across the entire stream, avoiding variations in field nomenclature that may arise from different systems or individuals. For example, the field "customer ID" should be used uniformly across all records within the stream, minimizing misinterpretations and promoting data integrity.
- Data Type Enforcement
Strict adherence to defined data types is essential to maintaining the integrity of the "habs stream." This safeguards against unexpected or erroneous data inputs. Systems must validate incoming data against the pre-defined data types, flagging any deviations. Failure to enforce data type constraints can lead to unexpected results during analysis. For example, entering a string value where a numeric value is expected within a transaction record could cause major errors in calculations, potentially leading to incorrect financial reporting.
- Temporal Consistency
Ensuring consistent temporal patterns is crucial for accurate analysis of trends and patterns in the "habs stream." This involves specifying consistent time intervals for data capture and recording, which guarantees uniformity in data collection. Inaccurate or inconsistent time stamps can distort the observed patterns and trends within the stream. A "habs stream" tracking website traffic, for instance, needs precise timestamps to effectively evaluate the traffic over time and pinpoint specific peaks or troughs.
Maintaining format consistency in a "habs stream" is crucial for accurate analysis and decision-making. Consistent data structures, field names, and data types enable reliable and consistent results from data analysis. Ignoring these factors can lead to flawed insights, missed opportunities, and ultimately, less effective use of the "habs stream" as an operational asset. The quality of the "habs stream" relies heavily on the adherence to robust and consistent formatting guidelines.
3. Real-time Analysis
Real-time analysis is intrinsically linked to a "habs stream" by virtue of the stream's inherent nature. A "habs stream" is defined by its continuous flow of data, requiring immediate processing for actionable insights. The ability to analyze data in real-time is crucial for extracting timely trends, identifying anomalies, and enabling dynamic adjustments within systems. Without real-time analysis, the value proposition of a "habs stream" diminishes significantly, rendering the continuous flow of data merely a historical record.
Consider financial markets. A "habs stream" capturing trading activity necessitates immediate analysis to detect unusual price fluctuations or trading patterns. Real-time analysis allows algorithms to respond instantaneously, potentially preventing significant losses. Similarly, in fraud detection, a "habs stream" of transaction data requires real-time analysis to flag suspicious activities, enabling immediate action to mitigate potential fraud. In social media monitoring, real-time analysis of sentiment or user engagement enables businesses to adapt their strategies rapidly, allowing adjustments to marketing campaigns and customer service in response to emerging trends.
The practical significance of this understanding lies in optimizing decision-making processes. Real-time analysis allows for proactive responses, rather than reactive measures. This translates into quicker adjustments in strategies, more effective resource allocation, and improved efficiency across various domains. The limitations of real-time analysis, such as data latency or the complexity of algorithms, should also be acknowledged, as they can impact the accuracy and reliability of the insights gleaned. Overcoming these challenges through robust infrastructure and sophisticated algorithms is crucial for achieving the full potential of a "habs stream" and its real-time analysis capabilities.
4. Pattern Identification
Pattern identification plays a critical role within a "habs stream." The continuous flow of data inherent in a "habs stream" necessitates the ability to discern patterns. These patterns reveal underlying trends, anomalies, and relationships within the data, enabling informed decision-making. The effectiveness of a "habs stream" hinges on the accuracy and efficiency of pattern identification, as it directly impacts the value derived from the data. Without the capacity to identify patterns, the stream becomes a mere collection of unconnected data points, losing its potential for insightful analysis.
Consider a "habs stream" monitoring online retail transactions. Identifying patterns in purchasing behavior, such as recurring purchases of specific items by certain customer demographics, can unveil insights into consumer preferences and facilitate targeted marketing campaigns. Similarly, within a financial "habs stream," identifying patterns in trading volume or price movements might reveal fraudulent activities or market trends. In healthcare, a "habs stream" monitoring patient data can unveil patterns indicative of emerging diseases or potential health risks, prompting early interventions and preventative measures. Effective pattern recognition is thus essential to unlocking the value embedded within the continuous data flow of a "habs stream." Examples illustrate how this process translates to actionable insights across multiple domains.
In summary, accurate and timely pattern identification within a "habs stream" is critical. It transforms raw data into meaningful insights. The ability to discern patterns enables proactive responses to emerging trends, prevents potential issues, and empowers data-driven decision-making. Challenges in pattern identification, such as the sheer volume of data or the complexity of patterns, necessitate sophisticated algorithms and robust analytical tools. Addressing these challenges is crucial for maximizing the benefits of a "habs stream" and its applications in diverse fields. The effectiveness of a "habs stream" ultimately rests on the power of identifying patterns within the constantly flowing data.
5. Predictive Modeling
Predictive modeling, when integrated with a "habs stream," leverages the continuous flow of data to anticipate future outcomes. This capability is significant because it enables proactive strategies, risk mitigation, and optimized resource allocation. The ability to forecast future trends based on current and historical data gleaned from the "habs stream" allows for more informed and timely decisions.
- Data Preparation and Feature Engineering
The quality of predictive models derived from a "habs stream" hinges on the meticulous preparation of the data. This includes cleaning, transforming, and selecting relevant features. Data quality assurance is crucial for avoiding errors and biases, ensuring the reliability of the predictive model's output. Feature engineering, which involves extracting relevant characteristics from the "habs stream" data, is a key step in this process. For example, converting raw transaction data into usable features, such as average transaction value or frequency, can be crucial in identifying patterns relevant to future financial performance.
- Model Selection and Training
Appropriate model selection is critical. The chosen model must align with the specific prediction task and the characteristics of the data within the "habs stream." Training the model involves feeding the prepared data to the selected algorithm. Effective model training ensures the algorithm learns from the data within the "habs stream" and establishes accurate predictive capabilities. Choosing the optimal algorithm for tasks like customer churn prediction or demand forecasting based on real-time data in the "habs stream" is vital.
- Model Evaluation and Refinement
Evaluating the model's performance against established metrics is essential. This step assesses the model's ability to accurately predict future outcomes. Refinement of the model based on evaluation results is necessary. Techniques for assessing and tuning the model, taking into account the specific characteristics of the "habs stream" data, help ensure the model remains relevant and accurate as the data in the stream evolves.
- Integration into Decision-Making Processes
Predictive models derived from a "habs stream" are most impactful when integrated into the decision-making cycle. The insights from these models can inform strategies, allocate resources effectively, and mitigate risks. Clear communication of model outputs and their limitations is paramount in translating data-driven insights into actionable strategies. Integrating these models into operational software systems and workflows ensures real-time application of predictive insights derived from the "habs stream."
In conclusion, the integration of predictive modeling with a "habs stream" offers a powerful approach to anticipating future trends and adapting strategies accordingly. A well-structured and implemented system for data preparation, model selection, evaluation, and integration will enable robust and actionable insights, ensuring the "habs stream" serves as a valuable tool for proactive decision-making.
6. Decision Support
Decision support, a critical component of a "habs stream," leverages the continuous flow of data to provide context for informed choices. The stream itself becomes a platform for generating insights that facilitate effective decision-making across various domains. The quality of decisions hinges on the depth and accuracy of the information the "habs stream" provides. A well-structured "habs stream," encompassing real-time data analysis and predictive modeling, equips decision-makers with the information necessary to proactively address issues and capitalize on opportunities.
The practical significance of this connection is evident in diverse applications. For instance, in financial markets, a "habs stream" tracking trading activities allows for real-time monitoring of market trends and anomalies. This data, processed quickly and analyzed effectively, enables traders and investment professionals to make informed decisions, potentially minimizing risk and maximizing returns. Similarly, in healthcare, a "habs stream" tracking patient data allows for early detection of emerging health risks, enabling timely interventions and optimized resource allocation. Moreover, in logistics, a "habs stream" monitoring supply chain processes identifies potential bottlenecks and inefficiencies, thereby streamlining operations and optimizing delivery schedules. These examples illustrate the transformative potential of a "habs stream" to support decisions across diverse sectors.
In essence, "decision support" is not merely an adjunct to a "habs stream," but integral to its purpose. A "habs stream," by its nature, demands a mechanism for interpreting and applying its insights. The ability to translate the constant flow of data into actionable intelligencein other words, decision supportis paramount. This necessitates sophisticated tools and frameworks for analysis, visualization, and decision-making support. Furthermore, careful consideration must be given to factors like data accuracy, potential biases, and the ethical implications of relying on such systems for critical choices. This intricate relationship between a "habs stream" and decision support ultimately shapes how organizations operate and respond to the ever-changing landscape around them. The successful implementation of a "habs stream" requires robust decision support mechanisms to maximize its value.
7. Scalability
The ability of a "habs stream" to adapt and expand its capacity to handle increasing volumes of data is critical to its long-term viability and effectiveness. Scalability directly impacts the stream's usefulness in managing growing datasets, ensuring ongoing performance and value even as data volumes increase over time. Understanding the facets of scalability in a "habs stream" is crucial for assessing its practical application across various domains.
- Data Volume Management
A "habs stream" must accommodate expanding data volumes without compromising speed or accuracy. This involves robust infrastructure capable of handling high-throughput data ingestion and processing. Scalability necessitates solutions that can adapt to exponential growth in data collection, ensuring the system does not become a bottleneck. For example, a "habs stream" tracking global financial transactions requires infrastructure capable of handling millions of transactions per second. Failure to address this scalability requirement could result in delays and errors in processing crucial data.
- Processing Capacity
The processing power needed to analyze the data within a "habs stream" must scale accordingly. Algorithms and analytical tools must be designed to handle increasing data complexity, ensuring accurate interpretation and pattern identification. Scaling processing capacity might involve distributing computations across multiple servers, implementing parallel processing techniques, or developing optimized algorithms. Efficient scaling of processing power ensures the stream can derive meaningful insights from increasing data volumes in real-time.
- Storage Capacity
Storage solutions must scale to accommodate the persistent growth of the "habs stream" data. This involves choosing appropriate storage technologies, ensuring efficient data archiving, and optimizing data retrieval processes. Efficient data storage is essential to avoid performance degradation and data loss when dealing with massive amounts of information. Examples include cloud storage solutions that allow for elastic expansion of storage space, accommodating growing data volumes with minimal disruption to the ongoing stream.
- System Architecture
A scalable architecture is fundamental to a "habs stream." Modular design, loose coupling between components, and flexible integration points are crucial for handling increasing data volumes. This facilitates the addition of new features and modules without significant disruptions. For example, a modular architecture allows for the addition of new data sources to the stream without impacting the existing ones. Scalability in the system architecture is critical to accommodate changing demands and maintain effective data flow and processing within the stream.
In conclusion, the scalability of a "habs stream" is not merely a desirable feature but a fundamental requirement for its effectiveness and sustained utility. The ability to adapt to increasing data volumes across different aspectsdata volume, processing power, storage, and system architecturedefines the strength of a "habs stream." Without scalability, the stream's value diminishes as it struggles to handle the growing demands of the data it's intended to manage, potentially leading to unreliable results and impractical applications.
Frequently Asked Questions about "Habs Stream"
This section addresses common inquiries regarding "habs stream" data. The following questions and answers provide clarification on key concepts, applications, and limitations of this type of continuous data flow.
Question 1: What is a "habs stream," and what distinguishes it from other data streams?
A "habs stream" represents a continuous flow of structured data, specifically designed for detailed analysis of a particular domain. What distinguishes it from other data streams is the inherent structure, consistent format, and real-time analysis capabilities. This focused approach to data collection and processing allows for the identification of trends, patterns, and anomalies. Other data streams may lack this structured and ongoing nature.
Question 2: What are the typical applications of a "habs stream"?
Applications vary widely. A "habs stream" might track financial transactions, monitor social media sentiment, collect patient data in healthcare, or analyze supply chain activity. The specific use cases depend on the type of information collected and the analysis techniques implemented.
Question 3: How does data quality impact the reliability of a "habs stream"?
Data quality is paramount. Inconsistent formats, incomplete data points, or errors in data collection can significantly impair the reliability of the "habs stream" and lead to inaccurate analysis. Ensuring data accuracy and consistency is critical for deriving meaningful insights.
Question 4: What are the essential components for a robust "habs stream" infrastructure?
A robust infrastructure requires scalable data collection methods, consistent formatting standards, real-time processing capabilities, efficient storage solutions, and algorithms for effective pattern identification and predictive modeling. Scalability is crucial to adapt to increasing data volumes.
Question 5: What are the potential limitations of a "habs stream" approach?
While powerful, "habs streams" are not without limitations. The sheer volume of data can pose challenges in processing and analysis. Ensuring data quality and handling potential biases within the data are also crucial considerations. Interpreting complex patterns in real-time can be computationally demanding.
Understanding the specifics of a "habs stream" and its components is essential to its successful implementation. This FAQ provides a foundational overview, but careful consideration of the specific context and application is crucial before deployment.
Moving forward, this article will delve deeper into the architecture and implementation of "habs stream" systems, examining various use cases in more detail.
Conclusion
This exploration of "habs stream" has illuminated the crucial role of structured, continuous data flows in contemporary decision-making. Key aspects examined include the importance of data collection methodology, the necessity of consistent data formatting, the need for real-time analysis capabilities, the potential for predictive modeling, the application for decision support, and the essential attribute of scalability. The analysis underscored how this type of data stream, when implemented effectively, can provide organizations with a powerful platform for understanding complex systems, optimizing processes, and anticipating future trends.
The insights gained from the examination of "habs stream" systems highlight a significant advancement in data-driven strategies. Moving forward, a deeper understanding and more sophisticated application of these techniques will be essential for staying competitive in rapidly evolving fields. Continued development of scalable architectures, innovative analytical tools, and rigorous data quality management processes are vital to harnessing the transformative potential embedded within these structured data streams.
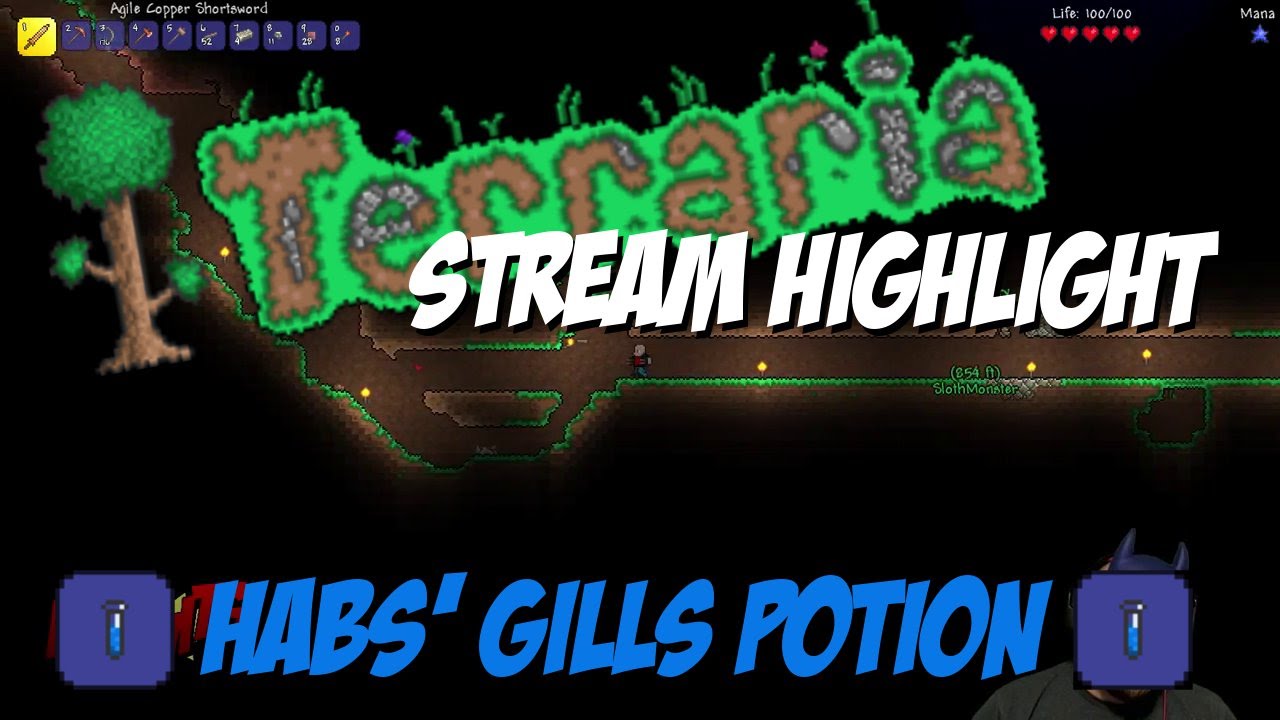
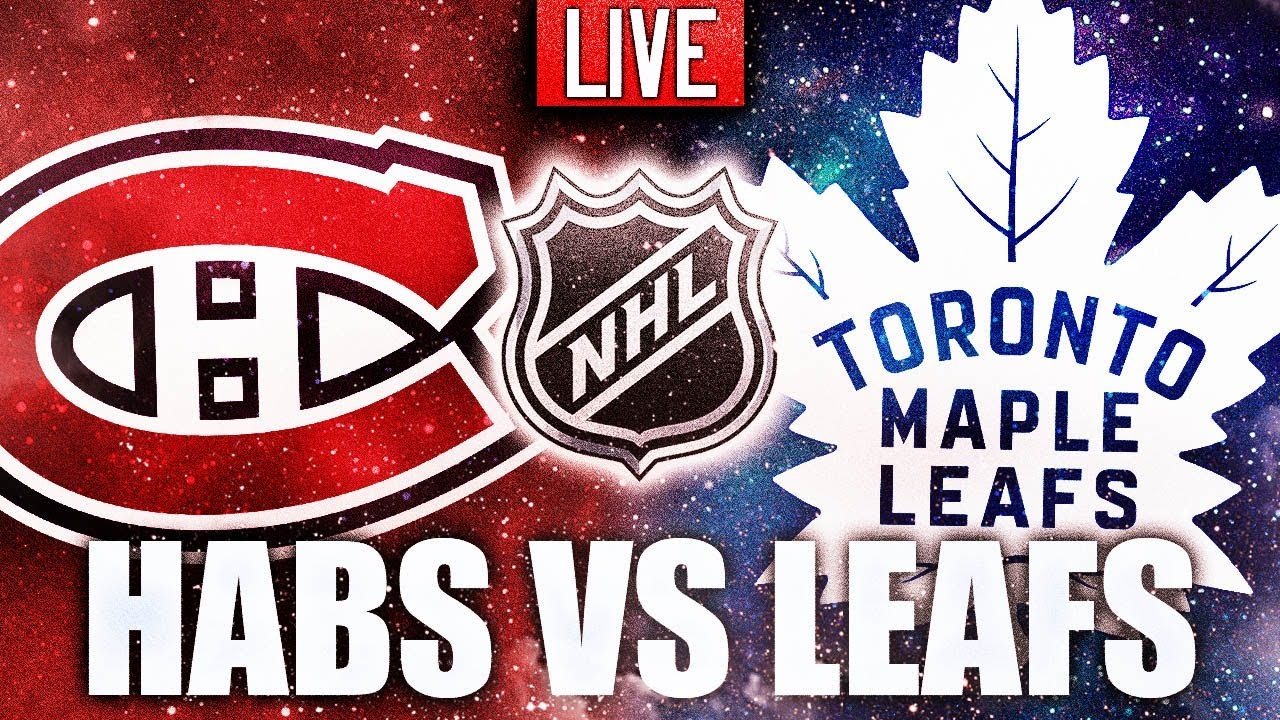
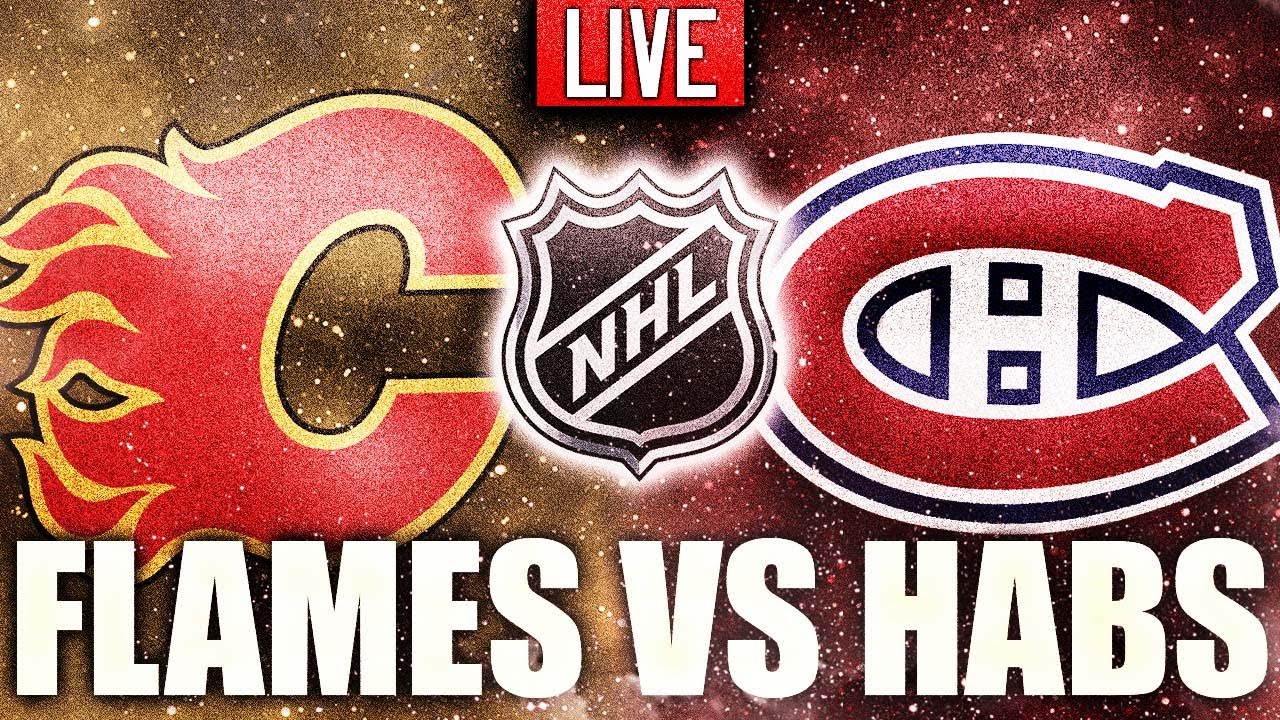
Detail Author:
- Name : Dr. Yoshiko Wiza
- Username : graham.myles
- Email : sanford.palma@sauer.com
- Birthdate : 1989-08-30
- Address : 8082 Rosina Wall Suite 829 Kemmerberg, AL 09785
- Phone : +13603628760
- Company : Nader-Howe
- Job : Paste-Up Worker
- Bio : In sit id ut consequatur aut deserunt porro. Aut molestiae aut ab est. Tempore molestiae necessitatibus tempore quos omnis.
Socials
tiktok:
- url : https://tiktok.com/@raul.kiehn
- username : raul.kiehn
- bio : Recusandae sint commodi dolore dolore atque.
- followers : 381
- following : 1731
instagram:
- url : https://instagram.com/raul_kiehn
- username : raul_kiehn
- bio : Qui magni velit consequatur nemo. Id dignissimos rerum velit nihil soluta.
- followers : 6922
- following : 537
twitter:
- url : https://twitter.com/rkiehn
- username : rkiehn
- bio : Quas autem et qui vel id quae. Est modi tenetur sed consectetur. Iure ut atque quis omnis fugiat.
- followers : 6643
- following : 30
linkedin:
- url : https://linkedin.com/in/raul_dev
- username : raul_dev
- bio : Voluptate exercitationem nihil velit odit.
- followers : 730
- following : 442
facebook:
- url : https://facebook.com/rkiehn
- username : rkiehn
- bio : Quam error voluptatem quia cupiditate et molestiae dolorum.
- followers : 6290
- following : 1532